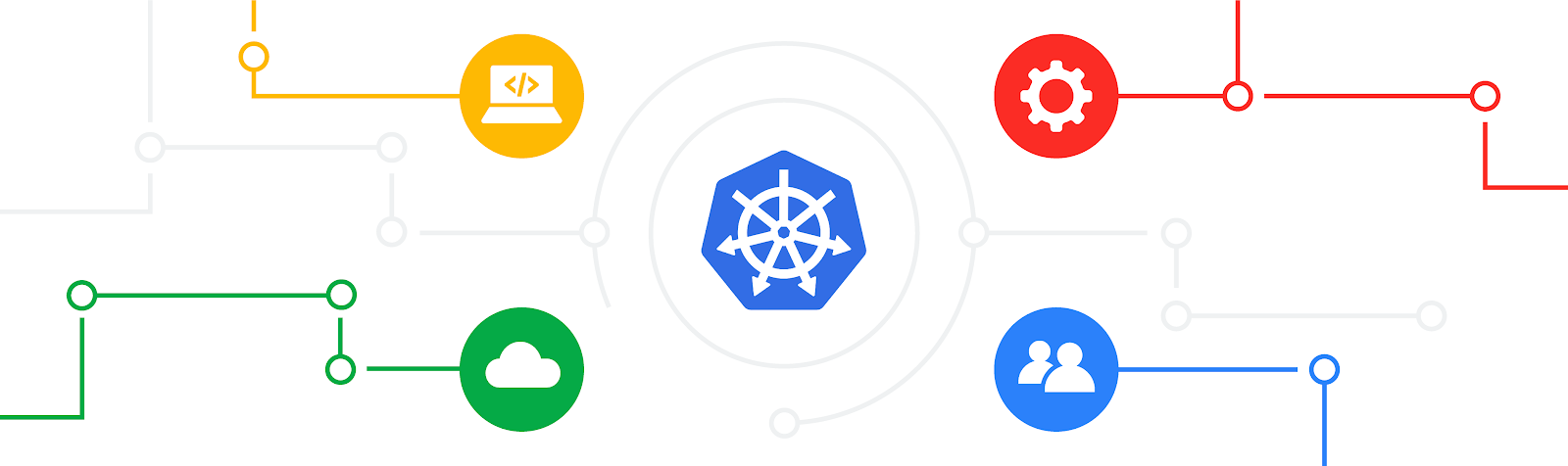
Kubernetes 1.33 is now available in the Google Kubernetes Engine (GKE) Rapid Channel! For more information about the content of Kubernetes 1.33, read the official Kubernetes 1.33 Release Notes and the specific GKE 1.33 Release Notes.
Enhancements in 1.33:
In-place Pod Resizing
Workloads can be scaled horizontally by updating the Pod replica count, or vertically by updating the resources required in the Pods container(s). Before this enhancement, container resources defined in a Pod's spec were immutable, and updating any of these details within a Pod template would trigger Pod replacement impacting service's reliability.
In-place Pod Resizing (IPPR, Public Preview) allows you to change the CPU and memory requests and limits assigned to containers within a running Pod through the new /resize pod subresource, often without requiring a container restart decreasing service's disruptions.
This opens up various possibilities for vertical scale-up of stateful processes without any downtime, seamless scale-down when the traffic is low, and even allocating larger resources during startup, which can then be reduced once the initial setup is complete.
Review Resize CPU and Memory Resources assigned to Containers for detailed guidance on using the new API.
DRA
Kubernetes Dynamic Resource Allocation (DRA), currently in beta as of v1.33, offers a more flexible API for requesting devices than Device Plugin. (Instructions for opt-in beta features in GKE)
Recent updates include the promotion of driver-owned resource claim status to beta. New alpha features introduced are partitionable devices, device taints and tolerations for managing device availability, prioritized device lists for versatile workload allocation, and enhanced admin access controls. Preparations for general availability include a new v1beta2 API to improve user experience and simplify future feature integration, alongside improved RBAC rules and support for seamless driver upgrades. DRA is anticipated to reach general availability in Kubernetes v1.34.
containerd 2.0
With GKE 1.33, we are excited to introduce support for containerd 2.0. This marks the first major version update for the underlying container runtime used by GKE. Adopting this version ensures that GKE continues to leverage the latest advancements and security enhancements from the upstream containerd community.
It's important to note that as a major version update, containerd 2.0 introduces many new features and enhancements while also deprecating others. To ensure a smooth transition and maintain compatibility for your workloads, we strongly encourage you to review your Cloud Recommendations. These recommendations will help identify any workloads that may be affected by these changes. Please see "Migrate nodes to containerd 2" for detailed guidance on making your workloads forward-compatible.
Multiple Service CIDRs
This enhancement introduced a new implementation of allocation logic for Service IPs. The updated IP address allocator logic uses two newly stable API objects: ServiceCIDR and IPAddress. Now generally available, these APIs allow cluster administrators to dynamically increase the number of IP addresses available for Services by creating new ServiceCIDR objects.
Highlight of Googlers' contributions in 1.33 cycle:
The Coordinated Leader Election feature progressed to beta, introducing significant enhancements in how a lease-candidate's availability is determined for an election. Specifically, the ping-acknowledgement checking process has been optimized to be fully concurrent instead of the previous sequential approach ensuring faster and more efficient detection of unresponsive candidates, which is essential for promptly identifying truly available lease candidates and maintaining the reliability of the leader election process.
New CLI flags were added to apiserver as options for adjusting API enablement wrt an apiserver's emulated version. --emulation-forward-compatible is an option to implicitly enable all APIs which are introduced after the emulation version and have higher priority than APIs of the same group resource enabled at the emulation version.
--runtime-config-emulation-forward-compatible is an option to explicit enable specific APIs introduced after the emulation version through the runtime-config
ComponentStatusz and ComponentFlagz alpha features are now available to be turned on for all control plane components.
Components now expose two new HTTP endpoints, /statusz and /flagz, providing enhanced visibility into their internal state. /statusz details the component's uptime, golang, binary and emulation versions info, while /flagz reveals the command-line arguments used at startup.
To improve cluster stability when handling large datasets, streaming encoding for List responses was introduced as a new Beta feature. Previously, serializing entire List responses into a single memory block could strain kube-apiserver memory. The new streaming encoder processes and transmits each item in a list individually, preventing large memory allocations. This significantly reduces memory spikes, improves API server reliability, and enhances overall cluster performance, especially for clusters with large resources, all while maintaining backward compatibility and requiring no client-side changes.
Further enhancing API server performance and stability, a new Alpha feature introduces snapshotting to the watchcache. This allows serving LIST requests for historical or paginated data directly from its in-memory cache. Previously, these types of requests would query etcd directly, requiring to pipe the data through multiple encoding, decoding, and validation stages. This process often led to increased memory pressure, unpredictable performance, and potential stability issues, especially with large resources. By leveraging efficient B-tree based snapshotting within the watchcache, this enhancement significantly reduces direct etcd load and minimizes memory allocations on the API server. This results in more predictable performance, increased API server reliability, and better overall resource utilization, while incorporating mechanisms to ensure data consistency between the cache and etcd.
Kubernetes thrives on its large, vibrant community of contributors. We're constantly looking for ways to help make it easier to maintain and contribute to this project. For years, one area that posed challenges was how the Kubernetes API itself was validated: using hand-written Go code. This traditional method has proven to be difficult to authors, challenging to review and cumbersome to document, impacting overall maintainability and the contributor experience. To address these pain points, the declarative validation project was initiated.
In 1.33, the foundational infrastructure was established to transition Kubernetes API validation from handwritten Go code to a declarative model using IDL tags. This release introduced the
validation-gen code generator, designed to parse these IDL tags and produce Go validation functions.
The current namespace deletion process is semi-random, which may lead to security gaps or unintended behavior, such as Pods persisting after the deletion of their associated NetworkPolicies. By implementing an opinionated deletion mechanism, the Pods will be deleted before other resources with respect to logical and security dependencies. This design enhances the security and reliability of Kubernetes by mitigating risks arising from the non-deterministic deletion order.
Acknowledgements
As always, we want to thank all the Googlers that provide their time, passion, talent and leadership to keep making Kubernetes the best container orchestration platform. We would like to mention especially Googlers who helped drive the contributions mentioned in this blog: Tim Allclair, Natasha Sarkar, Vivek Bansal, Anish Shah, Dawn Chen, Tim Hockin, John Belamaric, Morten Torkildsen, Yu Liao,Cici Huang, Samuel Karp, Chris Henzie, Luiz Oliveira, Piotr Betkier, Alex Curtis, Jonah Peretz, Brad Hoekstra, Yuhan Yao, Ray Wainman, Richa Banker, Marek Siarkowicz, Siyuan Zhang, Jeffrey Ying, Henry Wu, Yuchen Zhou, Jordan Liggitt, Benjamin Elder, Antonio Ojea, Yongrui Lin, Joe Betz, Aaron Prindle and the Googlers who helped bring 1.33 to GKE!
- Benjamin Elder & Sen Lu, Google Kubernetes Engine