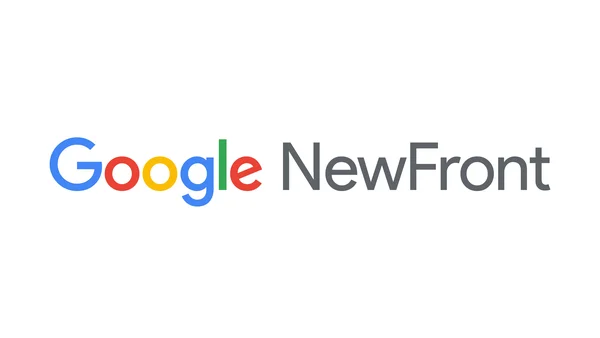
Display & Video 360 updates from Google NewFront
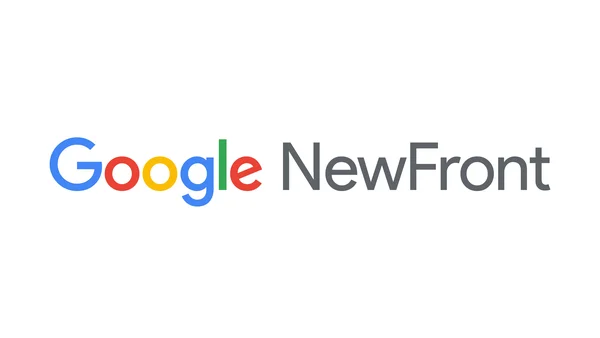
The Dev channel has been updated to 126.0.6439.0 for Windows, Mac and Linux.
A partial list of changes is available in the Git log. Interested in switching release channels? Find out how. If you find a new issue, please let us know by filing a bug. The community help forum is also a great place to reach out for help or learn about common issues.
Srinivas Sista
Google Chrome
The Dev channel has been updated to 126.0.6439.0 for Windows, Mac and Linux.
A partial list of changes is available in the Git log. Interested in switching release channels? Find out how. If you find a new issue, please let us know by filing a bug. The community help forum is also a great place to reach out for help or learn about common issues.
Srinivas Sista
Google Chrome
Hi everyone! We've just released Chrome Dev 126 (126.0.6437.4) for Android. It's now available on Google Play.
You can see a partial list of the changes in the Git log. For details on new features, check out the Chromium blog, and for details on web platform updates, check here.
If you find a new issue, please let us know by filing a bug.
Krishna Govind
Google Chrome