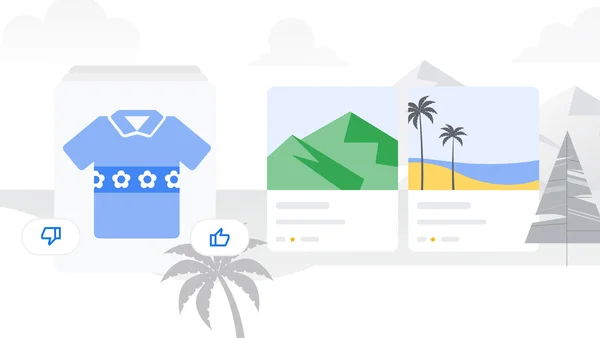
6 ways to travel smarter this summer using Google tools
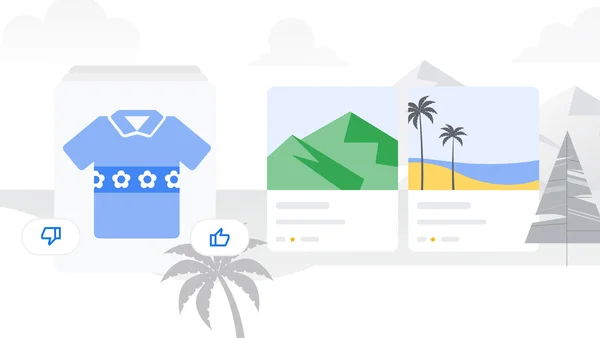
Speech-to-speech translation (S2ST) is a type of machine translation that converts spoken language from one language to another. This technology has the potential to break down language barriers and facilitate communication between people from different cultures and backgrounds.
Previously, we introduced Translatotron 1 and Translatotron 2, the first ever models that were able to directly translate speech between two languages. However they were trained in supervised settings with parallel speech data. The scarcity of parallel speech data is a major challenge in this field, so much that most public datasets are semi- or fully-synthesized from text. This adds additional hurdles to learning translation and reconstruction of speech attributes that are not represented in the text and are thus not reflected in the synthesized training data.
Here we present Translatotron 3, a novel unsupervised speech-to-speech translation architecture. In Translatotron 3, we show that it is possible to learn a speech-to-speech translation task from monolingual data alone. This method opens the door not only to translation between more language pairs but also towards translation of the non-textual speech attributes such as pauses, speaking rates, and speaker identity. Our method does not include any direct supervision to target languages and therefore we believe it is the right direction for paralinguistic characteristics (e.g., such as tone, emotion) of the source speech to be preserved across translation. To enable speech-to-speech translation, we use back-translation, which is a technique from unsupervised machine translation (UMT) where a synthetic translation of the source language is used to translate texts without bilingual text datasets. Experimental results in speech-to-speech translation tasks between Spanish and English show that Translatotron 3 outperforms a baseline cascade system.
Translatotron 3 addresses the problem of unsupervised S2ST, which can eliminate the requirement for bilingual speech datasets. To do this, Translatotron 3’s design incorporates three key aspects:
The model is trained using a combination of the unsupervised MUSE embedding loss, reconstruction loss, and S2S back-translation loss. During inference, the shared encoder is utilized to encode the input into a multilingual embedding space, which is subsequently decoded by the target language decoder.
Translatotron 3 employs a shared encoder to encode both the source and target languages. The decoder is composed of a linguistic decoder, an acoustic synthesizer (responsible for acoustic generation of the translation speech), and a singular attention module, like Translatotron 2. However, for Translatotron 3 there are two decoders, one for the source language and another for the target language. During training, we use monolingual speech-text datasets (i.e., these data are made up of speech-text pairs; they are not translations).
The encoder has the same architecture as the speech encoder in the Translatotron 2. The output of the encoder is split into two parts: the first part incorporates semantic information whereas the second part incorporates acoustic information. By using the MUSE loss, the first half of the output is trained to be the MUSE embeddings of the text of the input speech spectrogram. The latter half is updated without the MUSE loss. It is important to note that the same encoder is shared between source and target languages. Furthermore, the MUSE embedding is multilingual in nature. As a result, the encoder is able to learn a multilingual embedding space across source and target languages. This allows a more efficient and effective encoding of the input, as the encoder is able to encode speech from both languages into a common embedding space, rather than maintaining a separate embedding space for each language.
Like Translatotron 2, the decoder is composed of three distinct components, namely the linguistic decoder, the acoustic synthesizer, and the attention module. To effectively handle the different properties of the source and target languages, however, Translatotron 3 has two separate decoders, for the source and target languages.
The training methodology consists of two parts: (1) auto-encoding with reconstruction and (2) a back-translation term. In the first part, the network is trained to auto-encode the input to a multilingual embedding space using the MUSE loss and the reconstruction loss. This phase aims to ensure that the network generates meaningful multilingual representations. In the second part, the network is further trained to translate the input spectrogram by utilizing the back-translation loss. To mitigate the issue of catastrophic forgetting and enforcing the latent space to be multilingual, the MUSE loss and the reconstruction loss are also applied in this second part of training. To ensure that the encoder learns meaningful properties of the input, rather than simply reconstructing the input, we apply SpecAugment to encoder input at both phases. It has been shown to effectively improve the generalization capabilities of the encoder by augmenting the input data.
During the back-translation training phase (illustrated in the section below), the network is trained to translate the input spectrogram to the target language and then back to the source language. The goal of back-translation is to enforce the latent space to be multilingual. To achieve this, the following losses are applied:
In addition to these losses, SpecAugment is applied to the encoder input at both phases. Before the back-translation training phase, the network is trained to auto-encode the input to a multilingual embedding space using the MUSE loss and reconstruction loss.
To ensure that the encoder generates multilingual representations that are meaningful for both decoders, we employ a MUSE loss during training. The MUSE loss forces the encoder to generate such a representation by using pre-trained MUSE embeddings. During the training process, given an input text transcript, we extract the corresponding MUSE embeddings from the embeddings of the input language. The error between MUSE embeddings and the output vectors of the encoder is then minimized. Note that the encoder is indifferent to the language of the input during inference due to the multilingual nature of the embeddings.
![]() |
The training and inference in Translatotron 3. Training includes the reconstruction loss via the auto-encoding path and employs the reconstruction loss via back-translation. |
Following are examples of direct speech-to-speech translation from Translatotron 3:
Input (Spanish) | |
TTS-synthesized reference (English) | |
Translatotron 3 (English) |
Input (Spanish) | |
TTS-synthesized reference (English) | |
Translatotron 3 (English) |
Input (Spanish) | |
TTS reference (English) | |
Translatotron 3 (English) |
To empirically evaluate the performance of the proposed approach, we conducted experiments on English and Spanish using various datasets, including the Common Voice 11 dataset, as well as two synthesized datasets derived from the Conversational and Common Voice 11 datasets.
The translation quality was measured by BLEU (higher is better) on ASR (automatic speech recognition) transcriptions from the translated speech, compared to the corresponding reference translation text. Whereas, the speech quality is measured by the MOS score (higher is better). Furthermore, the speaker similarity is measured by the average cosine similarity (higher is better).
Because Translatotron 3 is an unsupervised method, as a baseline we used a cascaded S2ST system that is combined from ASR, unsupervised machine translation (UMT), and TTS (text-to-speech). Specifically, we employ UMT that uses the nearest neighbor in the embedding space in order to create the translation.
Translatotron 3 outperforms the baseline by large margins in every aspect we measured: translation quality, speaker similarity, and speech quality. It particularly excelled on the conversational corpus. Moreover, Translatotron 3 achieves speech naturalness similar to that of the ground truth audio samples (measured by MOS, higher is better).
![]() |
Translation quality (measured by BLEU, where higher is better) evaluated on three Spanish-English corpora. |
![]() |
Speech similarity (measured by average cosine similarity between input speaker and output speaker, where higher is better) evaluated on three Spanish-English corpora. |
![]() |
Mean-opinion-score (measured by average MOS metric, where higher is better) evaluated on three Spanish-English corpora. |
As future work, we would like to extend the work to more languages and investigate whether zero-shot S2ST can be applied with the back-translation technique. We would also like to examine the use of back-translation with different types of speech data, such as noisy speech and low-resource languages.
The direct contributors to this work include Eliya Nachmani, Alon Levkovitch, Yifan Ding, Chulayutsh Asawaroengchai, Heiga Zhen, and Michelle Tadmor Ramanovich. We also thank Yu Zhang, Yuma Koizumi, Soroosh Mariooryad, RJ Skerry-Ryan, Neil Zeghidour, Christian Frank, Marco Tagliasacchi, Nadav Bar, Benny Schlesinger and Yonghui Wu.
Last year our CEO, Sundar Pichai, announced that Google would invest $1 billion in Africa over the next five years to support a range of initiatives, from improved connectivity to investment in startups, to help boost Africa’s digital transformation.
Africa’s internet economy has the potential to grow to $180 billion by 2025 – 5.2% of the continent’s GDP. To support this growth, over the last year we’ve made progress on helping to enable affordable access and on building products for every African user – helping businesses build their online presence, supporting entrepreneurs spur next-generation technologies, and helping nonprofits to improve lives across the continent.
We’d like to share how we’re delivering on our commitment and partnering with others – policymakers, non-profits, businesses and creators – to make the internet more useful to more people in Africa.
Today we’re announcing our intent to establish a Google Cloud region in South Africa – our first on the continent. South Africa will be joining Google Cloud’s global network of 35 cloud regions and 106 zones worldwide.
The future cloud region in South Africa will bring Google Cloud services closer to our local customers, enabling them to innovate and securely deliver faster, more reliable experiences to their own customers, helping to accelerate their growth. According to research by AlphaBeta Economics for Google Cloud, the South Africa cloud region will contribute more than a cumulative USD 2.1 billion to the country’s GDP, and will support the creation of more than 40,000 jobs by 2030.
Niral Patel, Director for Cloud in Africa announces Google's intention to establish Google's first Cloud region in Africa
Along with the cloud region, we are expanding our network through the Equiano subsea cable and building Dedicated Cloud Interconnect sites in Johannesburg, Cape Town, Lagos and Nairobi. In doing so, we are building full scale Cloud capability for Africa.
We continue to support African entrepreneurs in growing their businesses and developing their talent. Our recently announced second cohort of the Black Founders Fund builds on the success of last year’s cohort, who raised $97 million in follow-on funding and have employed more than 500 additional staff since they were selected. We’re also continuing our support of African small businesses through the Hustle Academy and Google Business Profiles, and helping job seekers learn skills through Developer Scholarships and Career Certifications.
We’ve also continued to support nonprofits working to improve lives in Africa, with a $40 million cash and in-kind commitment so far. Over the last year this has included:
Across all our initiatives, we continue to work closely with our partners – most recently with the UN to launch the Global Africa Business Initiative (GABI), aimed at accelerating Africa’s economic growth and sustainable development.
We recently announced plans to open the first African product development centre in Nairobi. The centre will develop and build better products for Africans and the world.
Today, we’re launching voice typing support for nine more African languages (isiNdebele, isiXhosa, Kinyarwanda, Northern Sotho, Swati, Sesotho, Tswana, Tshivenda and Xitsonga) in Gboard, the Google keyboard – while 24 new languages are now supported on Google Translate, including Lingala, which is spoken by more than 45 million people across Central Africa.
To make Maps more useful, Street View imagery in Kenya, South Africa, Senegal and Nigeria has had a refresh with nearly 300,000 more kilometres of imagery now helping people virtually explore and navigate neighbourhoods. We’re also extending the service to Rwanda, meaning that Street View is now available in 11 African countries.
In addition to expanding the AI Accra Research Centre earlier this year, theOpen Buildings Project, which mapped buildings across the African continent using machine learning and satellite imagery, is expanding to South and Southeast Asia and is a great example of the AI centre creating solutions for Africa that are useful across the world.
We remain committed to working with our partners in building for Africa together, and helping to unlock the benefits of the digital economy for more people by providing useful products, programmes and investments. We’re doing this by partnering with African organisations, businesses and entrepreneurs. It’s the talent and drive of the individuals in the countries, communities and businesses of Africa that will power Africa’s economic growth.
Widgets are a simple way to personalize any Home screen, putting the information that’s most important to you — your inbox, the weather, your to-do list or even a photo of your dog — front and center.
With the upcoming launch of the nearby traffic widget for Google Maps (more on that soon), 35 Google widgets will be available on Android. To celebrate, we’re spotlighting five of our favorite widget features to help everyone better organize and personalize their Home screens.
Whether you’re commuting or heading out to meet friends, Google Maps’ real-time traffic predictions can help you easily plan your route. And with the new nearby traffic widget, launching in the coming weeks, you’ll see this information for your current location right from your Android Home screen. So if you're about to leave home, work, school or anywhere else, you’ll know at a glance exactly what local traffic might be like. And because Android widgets are tappable, you can zoom in and out without opening the Maps app.
The Gmail widget is a simple way to keep your inbox organized. Just tap to archive an email when it hits your inbox, without having to open the Gmail app.
Lots of you love the scrollable to-do list in the Keep widget. It’s an easy way to keep track of your tasks for the day, and there’s nothing quite as satisfying as crossing them off when you’re done — except maybe scrolling back up to see everything you accomplished.
The Google Translate widget’s scrolling feature can help you stay organized, too. If you need to keep certain phrases handy while you’re traveling or speaking with friends and family, you can set them up to always appear on your Home Screen. Just star your favorite translations in the app and you’ll see them right on the widget.
Android widgets are easy to resize and even change shape to help you declutter your Home screen — while keeping helpful features intact. For example, if you make the Drive widget smaller, it’ll turn into a toolbar so you can still quickly search for your files.
For years, Google Translate has helped break down language barriers and connect communities all over the world. And we want to make this possible for even more people — especially those whose languages aren’t represented in most technology. So today we’ve added 24 languages to Translate, now supporting a total of 133 used around the globe.
Over 300 million people speak these newly added languages — like Mizo, used by around 800,000 people in the far northeast of India, and Lingala, used by over 45 million people across Central Africa. As part of this update, Indigenous languages of the Americas (Quechua, Guarani and Aymara) and an English dialect (Sierra Leonean Krio) have also been added to Translate for the first time.
Translate's mission translated into some of our newly added languages
Here’s a complete list of the new languages now available in Google Translate:
This is also a technical milestone for Google Translate. These are the first languages we’ve added using Zero-Shot Machine Translation, where a machine learning model only sees monolingual text — meaning, it learns to translate into another language without ever seeing an example. While this technology is impressive, it isn't perfect. And we’ll keep improving these models to deliver the same experience you’re used to with a Spanish or German translation, for example. If you want to dig into the technical details, check out our Google AI blog post and research paper.
We’re grateful to the many native speakers, professors and linguists who worked with us on this latest update and kept us inspired with their passion and enthusiasm. If you want to help us support your language in a future update, contribute evaluations or translations through Translate Contribute.
When my wife and I were flying home from a trip to France a few years ago, our seatmate had just spent a few months exploring the French countryside and staying in small inns. When he learned that I worked on Google Translate, he got excited. He told me Translate’s conversation mode helped him chat with his hosts about family, politics, culture and more. Thanks to Translate, he said, he connected more deeply with people around him while in France.
One of the earliest versions of the Google Translate app for Android.
We released our Android app in January 2010, just over a year after the first commercial Android device was launched. As people started using the new Translate app over the next few years, we added a number of features to improve their experience, including early versions of conversation mode, offline translation and translating handwritten or printed text.
Our Android app crossed 100 million installs exactly four years after we first launched it. In 2014, Google acquired QuestVisual, the maker of WordLens. Together with the WordLens team, Translate’s goal was to introduce an advanced visual translation experience in our app. Within eight months, the team delivered the ability to instantly translate text using a phone camera, just as the app reached 200 million installs.
As it approached 300 million installs, Translate improved in two major ways. First, revamping Translate's conversation mode enabled two people to converse with each other despite speaking different languages, helping people in their everyday lives, as featured in the video From Syria to Canada.
Second, Google Translate's rollout of Neural Machine Translation, well underway when the app reached 500 million installs, greatly improved the fluency of our translations across text, speech and visual translation. As the installs continued to grow, we compressed those advanced models down to a size that can run on a phone. Offline translations made these high-quality translations available to anyone even when there is no network or connectivity is poor.
Transcribe, our long-form speech translation feature, launched when we reached 850 million installs. We partnered with the Pixel Buds team to offer streaming speech translations on top of our Transcribe feature, for more natural conversations between people speaking different languages. During this time, we improved the accuracy and increased the number of supported languages for offline translation.
Aside from these features, our engineering team has spent countless hours on bringing our users a simple-to-use experience on a stable app, keeping up with platform needs and rigorously testing changes before they launch. As we celebrate this milestone and all our users whose experiences make the work meaningful, we also celebrate our engineers who build with care, our designers who fret over every pixel and our product team who bring focus.
Our mission is to enable everyone, everywhere to understand the world and express themselves across languages. Looking beyond one billion installs, we’re looking forward to continually improving translation quality and user experiences, supporting more languages and helping everyone communicate, every day.
Recently, I was at my friend’s family gathering, where her grandmother told a story from her childhood. I could see that she was excited to share it with everyone but there was a problem—she told the story in Spanish, a language that I don’t understand. I pulled out Google Translate to transcribe the speech as it was happening. As she was telling the story, the English translation appeared on my phone so that I could follow along—it fostered a moment of understanding that would have otherwise been lost. And now anyone can do this—starting today, you can use the Google Translate Android app to transcribe foreign language speech as it’s happening.
Transcribe will be rolling out in the next few days with support for any combination of the following eight languages: English, French, German, Hindi, Portuguese, Russian, Spanish and Thai.
To try the transcribe feature, go to your Translate app on Android, and make sure you have the latest updates from the Play store. Tap on the “Transcribe” icon from the home screen and select the source and target languages from the language dropdown at the top. You can pause or restart transcription by tapping on the mic icon. You also can see the original transcript, change the text size or choose a dark theme in the settings menu.
On the left: redesigned home screen. On the right: how to change the settings for a comfortable read.
We’ll continue to make speech translations available in a variety of situations. Right now, the transcribe feature will work best in a quiet environment with one person speaking at a time. In other situations, the app will still do its best to provide the gist of what's being said. Conversation mode in the app will continue to help you to have a back and forth translated conversation with someone.
Try it out and give us feedback on how we can be better.
Recently, I was at my friend’s family gathering, where her grandmother told a story from her childhood. I could see that she was excited to share it with everyone but there was a problem—she told the story in Spanish, a language that I don’t understand. I pulled out Google Translate to transcribe the speech as it was happening. As she was telling the story, the English translation appeared on my phone so that I could follow along—it fostered a moment of understanding that would have otherwise been lost. And now anyone can do this—starting today, you can use the Google Translate Android app to transcribe foreign language speech as it’s happening.
Transcribe will be rolling out in the next few days with support for any combination of the following eight languages: English, French, German, Hindi, Portuguese, Russian, Spanish and Thai.
To try the transcribe feature, go to your Translate app on Android, and make sure you have the latest updates from the Play store. Tap on the “Transcribe” icon from the home screen and select the source and target languages from the language dropdown at the top. You can pause or restart transcription by tapping on the mic icon. You also can see the original transcript, change the text size or choose a dark theme in the settings menu.
On the left: redesigned home screen. On the right: how to change the settings for a comfortable read.
We’ll continue to make speech translations available in a variety of situations. Right now, the transcribe feature will work best in a quiet environment with one person speaking at a time. In other situations, the app will still do its best to provide the gist of what's being said. Conversation mode in the app will continue to help you to have a back and forth translated conversation with someone.
Try it out and give us feedback on how we can be better.