Posted by Guillaume Laforge, Developer Advocate for Google Cloud
With the beta of the new Java 11 runtime for Google Cloud Functions, Java developers can now write their functions using the Java programming language (a language often used in enterprises) in addition to Node.js, Go, or Python. Cloud Functions allow you to run bits of code locally or in the cloud, without provisioning or managing servers: Deploy your code, and let the platform handle scaling up and down for you. Just focus on your code: handle incoming HTTP requests or respond to some cloud events, like messages coming from Cloud Pub/Sub or new files uploaded in Cloud Storage buckets.
In this article, let’s focus on what functions look like, how you can write portable functions, how to run and debug them locally or deploy them in the cloud or on-premises, thanks to the Functions Framework, an open source library that runs your functions. But you will also learn about third-party frameworks that you might be familiar with, that also let you create functions using common programming paradigms.
The shape of your functions
There are two types of functions: HTTP functions, and background functions. HTTP functions respond to incoming HTTP requests, whereas background functions react to cloud-related events.
The Java Functions Framework provides an API that you can use to author your functions, as well as an invoker which can be called to run your functions locally on your machine, or anywhere with a Java 11 environment.
To get started with this API, you will need to add a dependency in your build files. If you use Maven, add the following dependency tag in pom.xml
:
<dependency>
<groupId>com.google.cloud.functions</groupId>
<artifactId>functions-framework-api</artifactId>
<version>1.0.1</version>
<scope>provided</scope>
</dependency>
If you are using Gradle, add this dependency declaration in build.gradle
:
compileOnly("com.google.cloud.functions:functions-framework-api")
Responding to HTTP requests
A Java function that receives an incoming HTTP request implements the HttpFunction
interface:
import com.google.cloud.functions.*;
import java.io.*;
public class Example implements HttpFunction {
@Override
public void service(HttpRequest request, HttpResponse response)
throws IOException {
var writer = response.getWriter();
writer.write("Hello developers!");
}
}
The service()
method provides an HttpRequest
and an HttpResponse
object. From the request, you can get information about the HTTP headers, the payload body, or the request parameters. It’s also possible to handle multipart requests. With the response, you can set a status code or headers, define a body payload and a content-type.
Responding to cloud events
Background functions respond to events coming from the cloud, like new Pub/Sub messages, Cloud Storage file updates, or new or updated data in Cloud Firestore. There are actually two ways to implement such functions, either by dealing with the JSON payloads representing those events, or by taking advantage of object marshalling thanks to the Gson library, which takes care of the parsing transparently for the developer.
With a RawBackgroundFunction, the responsibility is on you to handle the incoming cloud event JSON-encoded payload. You receive a JSON string, so you are free to parse it however you like, with your JSON parser of your choice:
import com.google.cloud.functions.Context;
import com.google.cloud.functions.RawBackgroundFunction;
public class RawFunction implements RawBackgroundFunction {
@Override
public void accept(String json, Context context) {
...
}
}
But you also have the option to write a BackgroundFunction which uses Gson for unmarshalling a JSON representation into a Java class (a POJO, Plain-Old-Java-Object) representing that payload. To that end, you have to provide the POJO as a generic argument:
import com.google.cloud.functions.Context;
import com.google.cloud.functions.BackgroundFunction;
public class PubSubFunction implements BackgroundFunction<PubSubMsg> {
@Override
public void accept(PubSubMsg msg, Context context) {
System.out.println("Received message ID: " + msg.messageId);
}
}
public class PubSubMsg {
String data;
Map<String, String> attributes;
String messageId;
String publishTime;
}
The Context parameter contains various metadata fields like timestamps, the type of events, and other attributes.
Which type of background function should you use? It depends on the control you need to have on the incoming payload, or if the Gson unmarshalling doesn’t fully fit your needs. But having the unmarshalling covered by the framework definitely streamlines the writing of your function.
Running your function locally
Coding is always great, but seeing your code actually running is even more rewarding. The Functions Framework comes with the API we used above, but also with an invoker tool that you can use to run functions locally. For improving developer productivity, having a direct and local feedback loop on your own computer makes it much more comfortable than deploying in the cloud for each change you make to your code.
With Maven
If you’re building your functions with Maven, you can install the Function Maven plugin in your pom.xml
:
<plugin>
<groupId>com.google.cloud.functions</groupId>
<artifactId>function-maven-plugin</artifactId>
<version>0.9.2</version>
<configuration>
<functionTarget>com.example.Example</functionTarget>
</configuration>
</plugin>
On the command-line, you can then run:
$ mvn function:run
You can pass extra parameters like --target
to define a different function to run (in case your project contains several functions), --port
to specify the port to listen to, or --classpath
to explicitly set the classpath needed by the function to run. These are the parameters of the underlying Invoker
class. However, to set these parameters via the Maven plugin, you’ll have to pass properties with -Drun.functionTarget=com.example.Example
and -Drun.port
.
With Gradle
With Gradle, there is no dedicated plugin, but it’s easy to configure build.gradle
to let you run functions.
First, define a dedicated configuration for the invoker:
configurations {
invoker
}
In the dependencies, add the Invoker library:
dependencies {
invoker 'com.google.cloud.functions.invoker:java-function-invoker:1.0.0-beta1'
}
And then, create a new task to run the Invoker:
tasks.register("runFunction", JavaExec) {
main = 'com.google.cloud.functions.invoker.runner.Invoker'
classpath(configurations.invoker)
inputs.files(configurations.runtimeClasspath,
sourceSets.main.output)
args('--target',
project.findProperty('runFunction.target') ?:
'com.example.Example',
'--port',
project.findProperty('runFunction.port') ?: 8080
)
doFirst {
args('--classpath', files(configurations.runtimeClasspath,
sourceSets.main.output).asPath)
}
}
By default, the above launches the function com.example.Example
on port 8080, but you can override those on the command-line, when running gradle or the gradle wrapper:
$ gradle runFunction -PrunFunction.target=com.example.HelloWorld \
-PrunFunction.port=8080
Running elsewhere, making your functions portable
What’s interesting about the Functions Framework is that you are not tied to the Cloud Functions platform for deploying your functions. As long as, in your target environment, you can run your functions with the Invoker class, you can run your functions on Cloud Run, on Google Kubernetes Engine, on Knative environments, on other clouds when you can run Java, or more generally on any servers on-premises. It makes your functions highly portable between environments. But let’s have a closer look at deployment now.
Deploying your functions
You can deploy functions with the Maven plugin as well, with various parameters to tweak for defining regions, memory size, etc. But here, we’ll focus on using the cloud SDK, with its gcloud
command-line, to deploy our functions.
For example, to deploy an HTTP function, you would type:
$ gcloud functions deploy exampleFn \
--region europe-west1 \
--trigger-http \
--allow-unauthenticated \
--runtime java11 \
--entry-point com.example.Example \
--memory 512MB
For a background function that would be notified of new messages on a Pub/Sub topic, you would launch:
$ gcloud functions deploy exampleFn \
--region europe-west1 \
--trigger-topic msg-topic \
--runtime java11 \
--entry-point com.example.PubSubFunction \
--memory 512MB
Note that deployments come in two flavors as well, although the above commands are the same: functions are deployed from source with a pom.xml
and built in Google Cloud, but when using a build tool other than Maven, you can also use the same command to deploy a pre-compiled JAR that contains your function implementation. Of course, you’ll have to create that JAR first.
What about other languages and frameworks?
So far, we looked at Java and the plain Functions Framework, but you can definitely use alternative JVM languages such as Apache Groovy, Kotlin, or Scala, and third-party frameworks that integrate with Cloud Functions like Micronaut and Spring Boot!
Pretty Groovy functions
Without covering all those combinations, let’s have a look at two examples. What would an HTTP function look like in Groovy?
The first step will be to add Apache Groovy as a dependency in your pom.xml
:
<dependency>
<groupId>org.codehaus.groovy</groupId>
<artifactId>groovy-all</artifactId>
<version>3.0.4</version>
<type>pom</type>
</dependency>
You will also need the GMaven compiler plugin to compile the Groovy code:
<plugin>
<groupId>org.codehaus.gmavenplus</groupId>
<artifactId>gmavenplus-plugin</artifactId>
<version>1.9.0</version>
<executions>
<execution>
<goals>
<goal>addSources</goal>
<goal>addTestSources</goal>
<goal>compile</goal>
<goal>compileTests</goal>
</goals>
</execution>
</executions>
</plugin>
When writing the function code, just use Groovy instead of Java:
import com.google.cloud.functions.*
class HelloWorldFunction implements HttpFunction {
void service(HttpRequest request, HttpResponse response) {
response.writer.write "Hello Groovy World!"
}
}
The same explanations regarding running your function locally or deploying it still applies: the Java platform is pretty open to alternative languages too! And the Cloud Functions builder will happily build your Groovy code in the cloud, since Maven lets you compile this code thanks to the Groovy library.
Micronaut functions
Third-party frameworks also offer a dedicated Cloud Functions integration. Let’s have a look at Micronaut.
Micronaut is a “modern, JVM-based, full-stack framework for building modular, easily testable microservice and serverless applications”, as explained on its website. It supports the notion of serverless functions, web apps and microservices, and has a dedicated integration for Google Cloud Functions.
In addition to being a very efficient framework with super fast startup times (which is important, to avoid long cold starts on serverless services), what’s interesting about using Micronaut is that you can use Micronaut’s own programming model, including Dependency Injection, annotation-driven bean declaration, etc.
For HTTP functions, you can use the framework’s own @Controller
/ @Get
annotations, instead of the Functions Framework’s own interfaces. So for example, a Micronaut HTTP function would look like:
import io.micronaut.http.annotation.*;
@Controller("/hello")
public class HelloController {
@Get(uri="/", produces="text/plain")
public String index() {
return "Example Response";
}
}
This is the standard way in Micronaut to define a Web microservice, but it transparently builds upon the Functions Framework to run this service as a Cloud Function. Furthermore, this programming model offered by Micronaut is portable across other environments, since Micronaut runs in many different contexts.
Last but not least, if you are using the Micronaut Launch project (hosted on Cloud Run) which allows you to scaffold new projects easily (from the command-line or from a nice UI), you can opt for adding the google-cloud-function
support module, and even choose your favorite language, build tool, or testing framework:
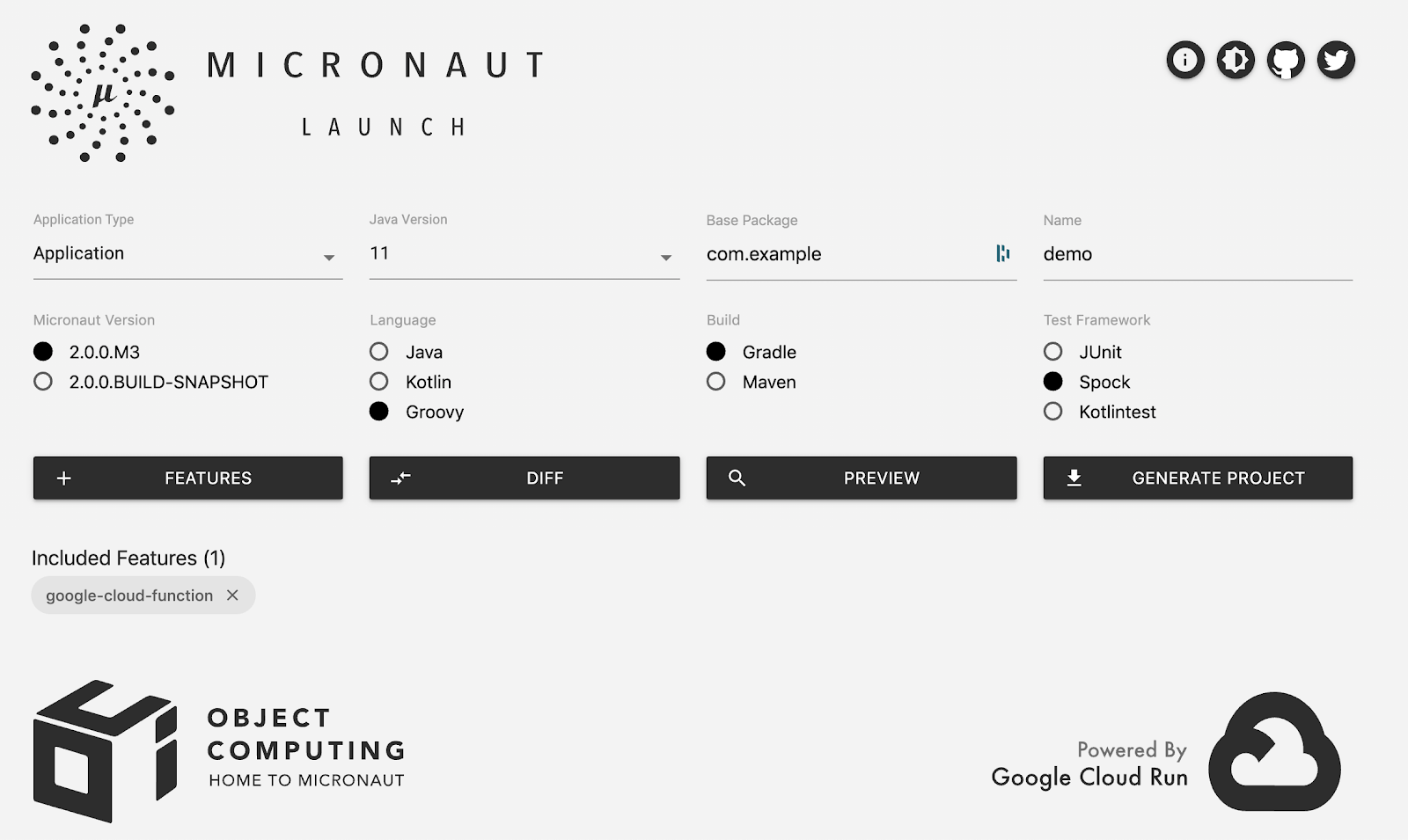
Be sure to check out the documentation for the Micronaut Cloud Functions support, and Spring Cloud Function support.
What’s next?
Now it’s your turn to try Cloud Functions for Java 11 today, with your favorite JVM language or third-party frameworks. Read the getting started guide, and try this for free with Google Cloud Platform free trial. Explore Cloud Functions’ features and use cases, take a look at the quickstarts, perhaps even contribute to the open source Functions Framework. And we’re looking forward to seeing what functions you’re going to build on this platform!