In this guest editorial, Patrick Brandt of The Coca-Cola Company tells us how they're using AI and TensorFlow to achieve frictionless proof-of-purchase. Coca-Cola's core loyalty program launched in 2006 as MyCokeRewards.com. The "MCR.com" platform included the creation of unique product codes for every Coca-Cola, Sprite, Fanta, and Powerade product sold in 20oz bottles and cardboard "fridge-packs" purchasable at grocery stores and other retail outlets. Users could enter these product codes at MyCokeRewards.com to participate in promotional campaigns.
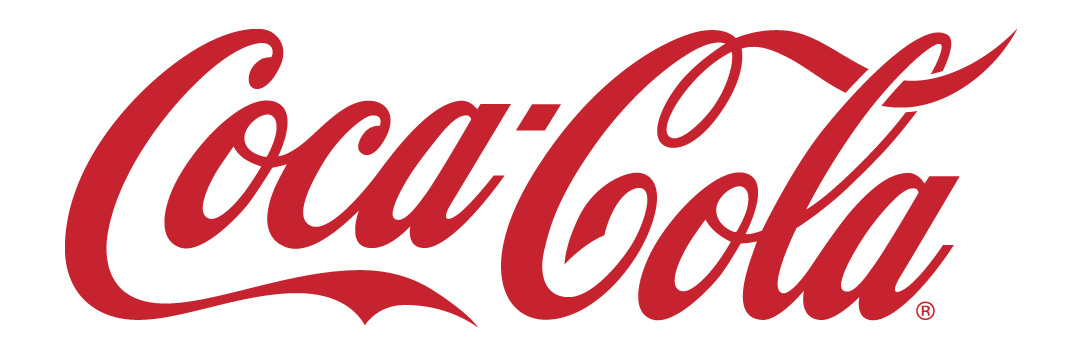
Fast-forward to 2016: Coke's loyalty programs are still hugely popular with millions of product codes having been entered for promotions and sweepstakes. However, mobile browsing went from non-existent in 2006 to over 50% share by the end of 2016. The launch of Coke.com as a mobile-first web experience (replacing MCR.com) was a response to these changes in browsing behavior. Thumb-entering 14-character codes into a mobile device could be a difficult enough user experience to impact the success of our programs. We want to provide our mobile audience the best possible experience, and recent advances in artificial intelligence opened new opportunities.
The quest for frictionless proof-of-purchase
For years Coke attempted to use off-the-shelf optical character recognition (OCR) libraries and services to read product codes with little success. Our printing process typically uses low-resolution dot-matrix fonts with the cap or fridge-pack media running under the printhead at very high speeds. All of this translates into a low-fidelity string of characters that defeats off-the-shelf OCR offerings (and can sometimes be hard to read with the human eye as well). OCR is critical to simplifying the code-entry process for mobile users: they should be able to take a picture of a code and automatically have the purchase registered for a promotional entry. We needed a purpose-built OCR system to recognize our product codes.
Bottlecap and fridge-pack examples
Our research led us to a promising solution: Convolutional Neural Networks. CNNs are one of a family of "deep learning" neural networks that are at the heart of modern artificial intelligence products. Google has used CNNs to extract street address numbers from StreetView images. CNNs also perform remarkably well at recognizing handwritten digits. These number-recognition use-cases were a perfect proxy for the type of problem we were trying to solve: extracting strings from images that contain small character sets with lots of variance in the appearance of the characters.
CNNs with TensorFlow
In the past, developing deep neural networks like CNNs has been a challenge because of the complexity of available training and inference libraries. TensorFlow, a machine learning framework that was open sourced by Google in November 2015, is designed to simplify the development of deep neural networks.
TensorFlow provides high-level interfaces to different kinds of neuron layers and popular loss functions, which makes it easier to implement different CNN model architectures. The ability to rapidly iterate over different model architectures dramatically reduced the time required to build Coke's custom OCR solution because different models could be developed, trained, and tested in a matter of days. TensorFlow models are also portable: the framework supports model execution natively on mobile devices ("AI on the edge") or in servers hosted remotely in the cloud. This enables a "create once, run anywhere" approach for model execution across many different platforms, including web-based and mobile.
Machine learning: practice makes perfect
Any neural network is only as good as the data used to train it. We knew that we needed a large set of labeled product-code images to train a CNN that would achieve our performance goals. Our training set would be built in three phases:
- Pre-launch simulated images
- Pre-launch real-world images
- Images labeled by our users in production
The pre-launch training phase began by programmatically generating millions of simulated product-code images. These simulated images included variations in tilt, lighting, shadows, and blurriness. The prediction accuracy (i.e. how often all 14 characters were correctly predicted within the top-10 predictions) was at 50% against real-world images when the model was trained using only simulated images. This provided a baseline for transfer-learning: a model initially trained with simulated images was the foundation for a more accurate model that would be trained against real-world images.
The challenge now turned to enriching the simulated images with enough real-world images to hit our performance goals. We created a purpose-built training app for iOS and Android devices that "trainers" could use to take pictures of codes and label them; these labeled images were then transferred to cloud storage for training. We did a production run of several thousand product codes on bottle caps and fridge-packs and distributed these to multiple suppliers who used the app to create the initial real-world training set.
Even with an augmented and enriched training set, there is no substitute for images created by end-users in a variety of environmental conditions. We knew that scans would sometimes result in an inaccurate code prediction, so we needed to provide a user-experience that would allow users to quickly correct these predictions. Two components are essential to delivering this experience: a product-code validation service that has been in use since the launch of our original loyalty platform in 2006 (to verify that a predicted code is an actual code) and a prediction algorithm that performs a regression to determine a per-character confidence at each one of the 14 character positions. If a predicted code is invalid, the top prediction as well as the confidence levels for each character are returned to the user interface. Low-confidence characters are visually highlighted to guide the user to update characters that need attention.
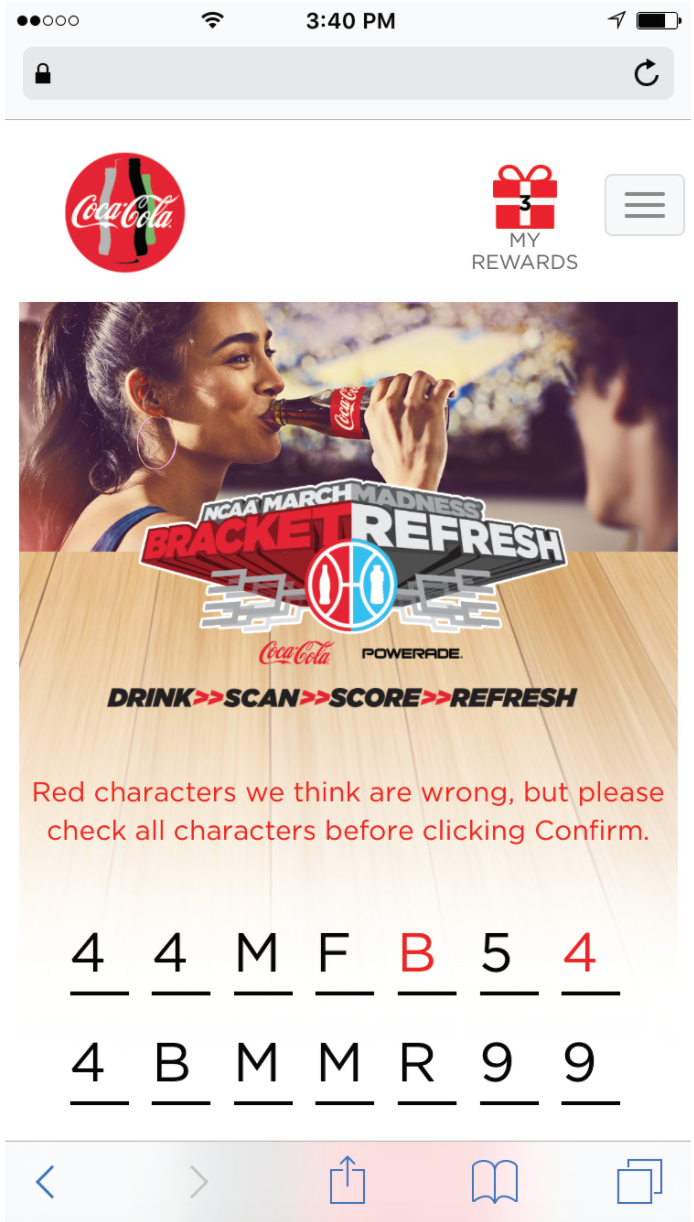
Error correction user interface lets users correct invalid predictions and generate useful training data
This user interface innovation enables an active learning process: a feedback loop allows the model to gradually improve by returning corrected predictions to the training pipeline. In this way, our users organically improve the accuracy of the character recognition model over time.
Product-code recognition pipeline
Optimizing for maximum performance
To meet user expectations around performance, we established a few ambitious requirements for the product-code OCR pipeline:
- It had to be fast: we needed a one-second average processing time once the image of the product-code was sent into the OCR pipeline
- It had to be accurate: our goal was to achieve 95% string recognition accuracy at launch with the guarantee that the model could be improved over time via active learning
- It had to be small: the OCR pipeline needs to be small enough to be distributed directly to mobile apps and accommodate over-the-air updates as the model improves over time
- It had to handle diverse product code media: dozens of different combinations of font types, bottlecaps, and cardboard fridge-pack media
We initially explored an architecture that used a single CNN for all product-code media. This approach created a model that was too large to be distributed to mobile apps and the execution time was longer than desired. Our applied-AI partners at Quantiphi, Inc.began iterating on different model architectures, eventually landing on one that used multiple CNNs.
This new architecture reduced the model size dramatically without sacrificing accuracy, but it was still on the high end of what we needed in order to support over-the-air updates to mobile apps. We next used TensorFlow's prebuilt quantization module to reduce the model size by reducing the fidelity of the weights between connected neurons. Quantization reduced the model size by a factor of 4, but a dramatic reduction in model size occurred when Quantiphi had a breakthrough using a new approach called SqueezeNet.
The SqueezeNet model was published by a team of researchers from UC Berkeley and Stanford in November of 2016. It uses a small but highly complex design to achieve accuracy levels on par with much larger models against popular benchmarks such as Imagenet. After re-architecting our character recognition models to use a SqueezeNet CNN, Quantiphi was able to reduce the model size of certain media types by a factor of 100. Since the SqueezeNet model was inherently smaller, a richer feature detection architecture could be constructed, achieving much higher accuracy at much smaller sizes compared to our first batch of models trained without SqueezeNet. We now have a highly accurate model that can be easily updated on remote devices; the recognition success rate of our final model before active learning was close to 96%, which translates into a 99.7% character recognition accuracy (just 3 misses for every 1000 character predictions).
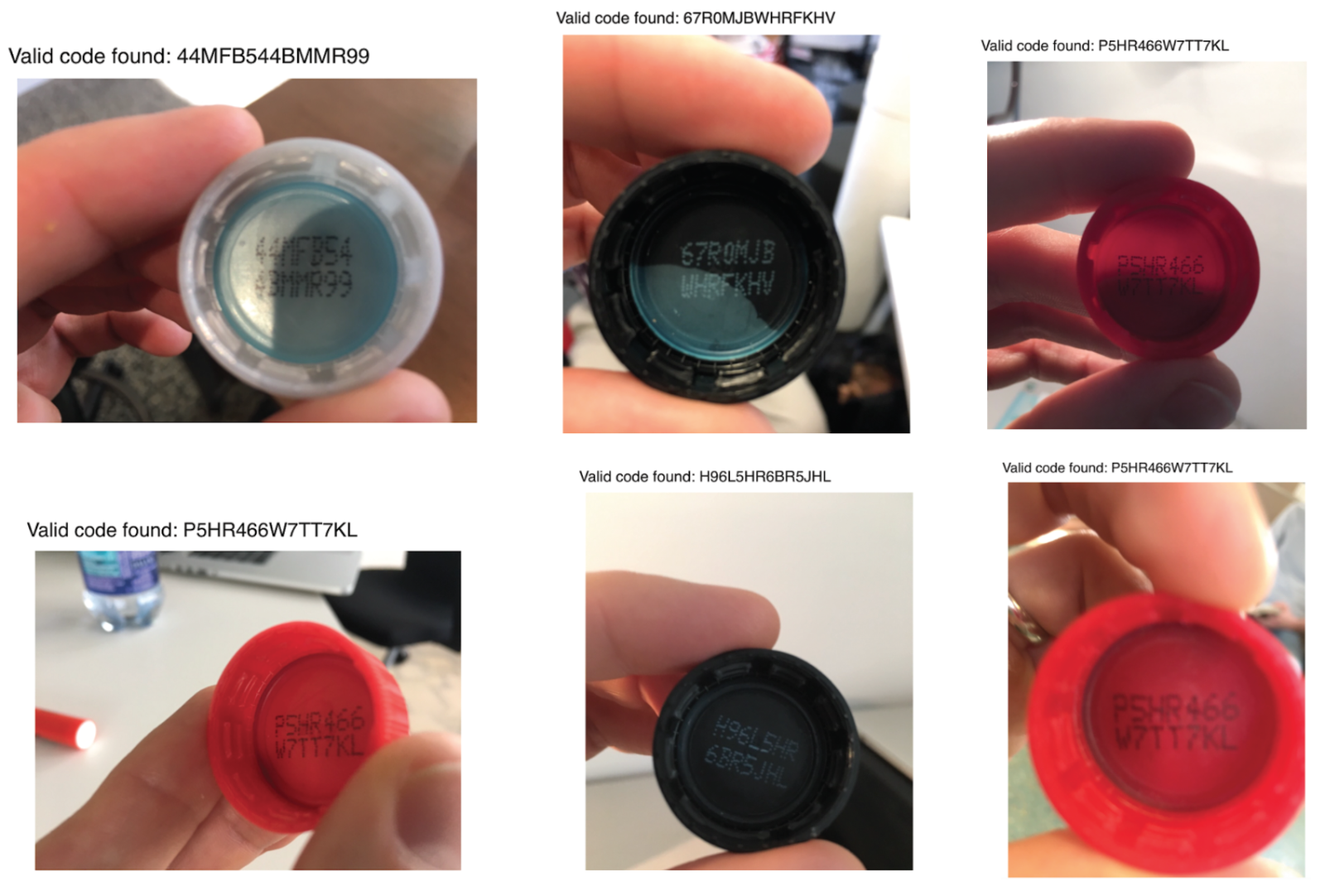
Valid product-code recognition examples with different types of occlusion, translation, and camera focus issues
Crossing boundaries with AI
Advances in artificial intelligence and the maturity of TensorFlow enabled us to finally achieve a long-sought proof-of-purchase capability. Since launching in late February 2017, our product code recognition platform has fueled more than a dozen promotions and resulted in over 180,000 scanned codes; it is now a core component for all of Coca-Cola North America's web-based promotions.
Moving to an AI-enabled product-code recognition platform has been valuable for two key reasons:
- Frictionless proof-of-purchase was enabled in a timely fashion, corresponding to our overall move to a mobile-first marketing platform.
- Coke saved millions of dollars by avoiding the requirement to update printers in our production lines to support higher-fidelity fonts that would work with existing off-the-shelf OCR software.
Our product-code recognition platform is the first execution of new AI-enabled capabilities at scale within Coca-Cola. We're now exploring AI applications across multiple lines of business, from new product development to ecommerce retail optimization.