Posted by Andrew Carroll, Product Lead, and Pi-Chuan Chang, Technical Lead, Google Health Sequencing genomes involves sampling short pieces of the DNA from the ~6 billion pairs of nucleobases — i.e., adenine (A), thymine (T), guanine (G), and cytosine (C) — we inherit from our parents. Genome sequencing is enabled by two key technologies: DNA sequencers (hardware) that "read" relatively small fragments of DNA, and variant callers (software) that combine the reads to identify where and how an individual's genome differs from a reference genome, like the one assembled in the Human Genome Project. Such variants may be indicators of genetic disorders, such as an elevated risk for breast cancer, pulmonary arterial hypertension, or neurodevelopmental disorders.
In 2017, we released DeepVariant, an open-source tool which identifies genome variants in sequencing data using a convolutional neural network (CNN). The sequencing process begins with a physical sample being sequenced by any of a handful of instruments, depending on the end goal of the sequencing. The raw data, which consists of numerous reads of overlapping fragments of the genome, are then mapped to a reference genome. DeepVariant analyzes these mappings to identify variant locations and distinguish them from sequencing errors.
Soon after it was first published in 2018, DeepVariant underwent a number of updates and improvements, including significant changes to improve accuracy for whole exome sequencing and polymerase chain reaction (PCR) sequencing.
We are now releasing DeepVariant v1.0, which incorporates a large number of improvements for all sequencing types. DeepVariant v1.0 is an improved version of our submission to the PrecisionFDA v2 Truth Challenge, which achieved Best Overall accuracy for 3 of 4 instrument categories. Compared to previous state-of-the-art models, DeepVariant v1.0 significantly reduces the errors for widely-used sequencing data types, including Illumina and Pacific Biosciences. In addition, through a collaboration with the UCSC Genomics Institute, we have also released a model that combines DeepVariant with the UCSC’s PEPPER method, called PEPPER-DeepVariant, which extends coverage to Oxford Nanopore data for the first time.
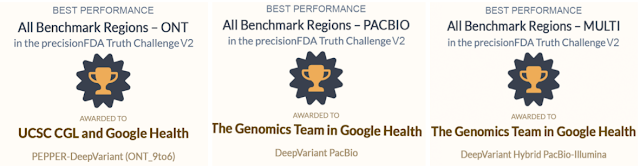
Sequencing Technologies and DeepVariant
For the last decade, the majority of sequence data were generated using Illumina instruments, which produce short (75-250 bases) and accurate sequences. In recent years, new technologies have become available that can sequence much longer pieces, including Pacific Biosciences, which can produce long and accurate sequences up to ~15,000 bases in length, and Oxford Nanopore, which can produce reads up to 1 million bases long, but with higher error rates. The particular type of sequencing data a researcher might use depends on the ultimate use-case.
Because DeepVariant is a deep learning method, we can quickly re-train it for these new instrument types, ensuring highly accurate sequence identification. Accuracy is important because a missed variant call could mean missing the causal variant for a disorder, while a false positive variant call could lead to identifying an incorrect one. Earlier state-of-the-art methods could reach ~99.1% accuracy (~73,000 errors) on a 35-fold coverage Illumina whole genome, whereas an early version of DeepVariant (v0.10) had ~99.4% accuracy (46,000 errors), corresponding to a 38% error reduction. DeepVariant v1.0 reduces Illumina errors by another ~22% and PacBio errors by another ~52% relative to the last DeepVariant release (v0.10).
DeepVariant Overview
DeepVariant is a convolutional neural network (CNN) that treats the task of identifying genetic variants as an image classification problem. DeepVariant constructs tensors, essentially multi-channel images, where each channel represents an aspect of the sequence, such as the bases in the sequence (called read base), the quality of alignment between different reads (mapping quality), whether a given read supports an alternate allele (read supports variant), etc. It then analyzes these data and outputs three genotype likelihoods, corresponding to how many copies (0, 1, or 2) of a given alternate allele are present.
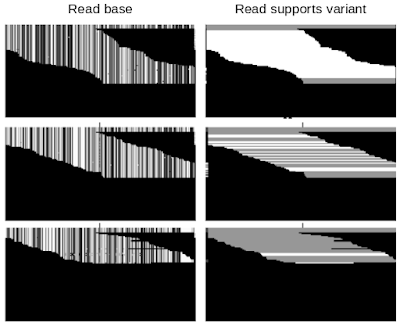 |
Example of DeepVariant data. Each row of pixels in each panel corresponds to a single read, i.e., a short genetic sequence. The top, middle, and bottom rows of panels present examples with a different number of variant alleles. Only two of the six data channels are shown: Read base — the pixel value is mapped to each of the four bases, A, C, G, or T; Read supports variant — white means that the read is consistent with a given allele and grey means it is not. Top: Classified by DeepVariant as a "2", which means that both chromosomes match the variant allele. Middle: Classified as a “1”, meaning that one chromosome matches the variant allele. Bottom: Classified as a “0”, implying that the variant allele is missing from both chromosomes. |
Technical Improvements in DeepVariant v1.0
Because DeepVariant uses the same codebase for each data type, improvements apply to each of Illumina, PacBio, and Oxford Nanopore. Below, we show the numbers for Illumina and PacBio for two types of small variants: SNPs (single nucleotide polymorphisms, which change a single base without changing sequence length) and INDELs (insertions and deletions).
- Training on an extended truth set
The Genome in a Bottle consortium from the National Institute of Standards and Technology (NIST) creates gold-standard samples with known variants covering the regions of the genome. These are used as labels to train DeepVariant. Using long-read technologies the Genome in a Bottle expanded the set of confident variants, increasing the regions described by the standard set from 85% of the genome to 92% of it. These more difficult regions were already used in training the PacBio models, and including them in the Illumina models reduced errors by 11%. By relaxing the filter for reads of lower mapping quality, we further reduced errors by 4% for Illumina and 13% for PacBio.
- Haplotype sorting of long reads
We inherit one copy of DNA from our mother and another from our father. PacBio and Oxford Nanopore sequences are long enough to separate sequences by parental origin, which is called a haplotype. By providing this information to the neural network, DeepVariant improves its identification of random sequence errors and can better determine whether a variant has a copy from one or both parents.
- Re-aligning reads to the alternate (ALT) allele
DeepVariant uses input sequence fragments that have been aligned to a reference genome. The optimal alignment for variants that include insertions or deletions could be different if the aligner knew they were present. To capture this information, we implemented an additional alignment step relative to the candidate variant. The figure below shows an additional second row where the reads are aligned to the candidate variant, which is a large insertion. You can see sequences that abruptly stop in the first row can now be fully aligned, providing additional information.
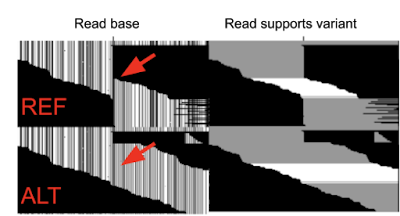 |
Example of DeepVariant data with realignment to ALT allele. DeepVariant is presented the information in both rows of data for the same example. Only two of the six data channels are shown: Read base (channel #1) and Read supports variant (channel #5). Top: Shows the reads aligned to the reference (in DeepVariant v0.10 and earlier this is all DeepVariant sees). Bottom: Shows the reads aligned to the candidate variant, in this case a long insertion of sequence). The red arrow indicates where the inserted sequence begins. |
- Use a small network to post-process outputs
Variants can have multiple alleles, with a different base inherited from each parent. DeepVariant’s classifier only generates a probability for one potential variant at a time. In previous versions, simple hand-written rules converted the probabilities into a composite call, but these rules failed in some edge cases. In addition, it also separated the way a final call was made from the backpropagation to train the network. By adding a small, fully-connected neural network to the post-processing step, we are able to better handle these tricky multi-allelic cases.
- Adding data to train the release model
The timeframe for the competition was compressed, so we trained only with data similar to the challenge data (PCR-Free NovaSeq) to speed model training. In our production releases, we seek high accuracy for multiple instruments as well as PCR+ preparations. Training with data from these diverse classes helps the model generalize, so our DeepVariant v1.0 release model outperforms the one submitted.
The charts below show the error reduction achieved by each improvement.
Training a Hybrid model
DeepVariant v1.0 also includes a hybrid model for PacBio and Illumina reads. In this case, the model leverages the strengths of both input types, without needing new logic.
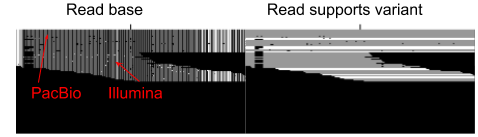 |
Example of DeepVariant merging data from both PacBio and Illumina. Only two of the six data channels are shown: Read base (channel #1) and Read supports variant (channel #5). The longer PacBio reads (at the upper part of the image) span the region being called entirely, while the shorter Illumin reads span only a portion of the region. |
We observed no change in SNP errors, suggesting that PacBio reads are strictly superior for SNP calling. We observed a further 49% reduction in Indel errors relative to the PacBio model, suggesting that the Indel error modes of Illumina and PacBio HiFi can be used in a complementary manner.
PEPPER-Deepvariant: A Pipeline for Oxford Nanopore Data Using DeepVariant
Until the PrecisionFDA competition, a DeepVariant model was not available for Oxford Nanopore data, because the higher base error rate created too many candidates for DeepVariant to classify. We partnered with the UC Santa Cruz Genomics Institute, which has extensive expertise with Nanopore data. They had previously trained a deep learning method called PEPPER, which could narrow down the candidates to a more tractable number. The larger neural network of DeepVariant can then accurately characterize the remaining candidates with a reasonable runtime.
The combined PEPPER-DeepVariant pipeline with the Oxford Nanopore model is open-source and available on GitHub. This pipeline was able to achieve a superior SNP calling accuracy to DeepVariant Illumina on the PrecisionFDA challenge, which is the first time anyone has shown Nanopore outperforming Illumina in this way.
Conclusion
DeepVariant v1.0 isn’t the end of development. We look forward to working with the genomics community to further maximize the value of genomic data to patients and researchers.