Posted by Jeremy Maitin-Shepard and Laramie Leavitt, Software Engineers, Connectomics at Google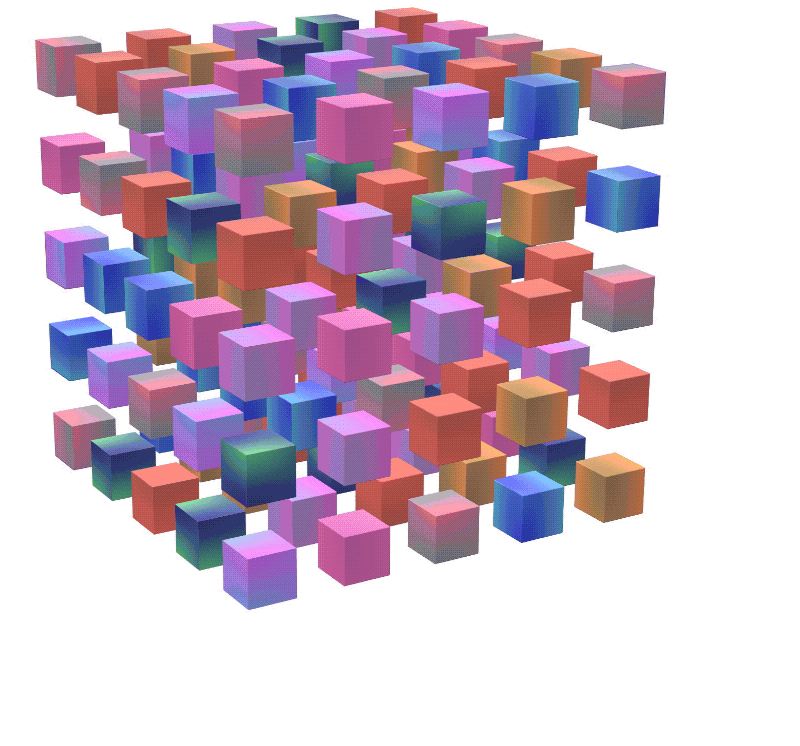
Many exciting contemporary applications of computer science and machine learning (ML) manipulate multidimensional datasets that span a single large coordinate system, for example, weather modeling from atmospheric measurements over a spatial grid or medical imaging predictions from multi-channel image intensity values in a 2d or 3d scan. In these settings, even a single dataset may require terabytes or petabytes of data storage. Such datasets are also challenging to work with as users may read and write data at irregular intervals and varying scales, and are often interested in performing analyses using numerous machines working in parallel.
Today we are introducing TensorStore, an open-source C++ and Python software library designed for storage and manipulation of n-dimensional data that:
- Provides a uniform API for reading and writing multiple array formats, including zarr and N5.
- Natively supports multiple storage systems, including Google Cloud Storage, local and network filesystems, HTTP servers, and in-memory storage.
- Supports read/writeback caching and transactions, with strong atomicity, isolation, consistency, and durability (ACID) guarantees.
- Supports safe, efficient access from multiple processes and machines via optimistic concurrency.
- Offers an asynchronous API to enable high-throughput access even to high-latency remote storage.
- Provides advanced, fully composable indexing operations and virtual views.
TensorStore has already been used to solve key engineering challenges in scientific computing (e.g., management and processing of large datasets in neuroscience, such as peta-scale 3d electron microscopy data and “4d” videos of neuronal activity). TensorStore has also been used in the creation of large-scale machine learning models such as PaLM by addressing the problem of managing model parameters (checkpoints) during distributed training.
Familiar API for Data Access and Manipulation
TensorStore provides a simple Python API for loading and manipulating large array data. In the following example, we create a TensorStore object that represents a 56 trillion voxel 3d image of a fly brain and access a small 100x100 patch of the data as a NumPy array:
>>> import tensorstore as ts
>>> import numpy as np
# Create a TensorStore object to work with fly brain data.
>>> dataset = ts.open({
... 'driver':
... 'neuroglancer_precomputed',
... 'kvstore':
... 'gs://neuroglancer-janelia-flyem-hemibrain/v1.1/segmentation/',
... }).result()
# Create a 3-d view (remove singleton 'channel' dimension):
>>> dataset_3d = dataset[ts.d['channel'][0]]
>>> dataset_3d.domain
{ "x": [0, 34432), "y": [0, 39552), "z": [0, 41408) }
# Convert a 100x100x1 slice of the data to a numpy ndarray
>>> slice = np.array(dataset_3d[15000:15100, 15000:15100, 20000])
Crucially, no actual data is accessed or stored in memory until the specific 100x100 slice is requested; hence arbitrarily large underlying datasets can be loaded and manipulated without having to store the entire dataset in memory, using indexing and manipulation syntax largely identical to standard NumPy operations. TensorStore also provides extensive support for advanced indexing features, including transforms, alignment, broadcasting, and virtual views (data type conversion, downsampling, lazily on-the-fly generated arrays).
The following example demonstrates how TensorStore can be used to create a zarr array, and how its asynchronous API enables higher throughput:
>>> import tensorstore as ts
>>> import numpy as np
>>> # Create a zarr array on the local filesystem
>>> dataset = ts.open({
... 'driver': 'zarr',
... 'kvstore': 'file:///tmp/my_dataset/',
... },
... dtype=ts.uint32,
... chunk_layout=ts.ChunkLayout(chunk_shape=[256, 256, 1]),
... create=True,
... shape=[5000, 6000, 7000]).result()
>>> # Create two numpy arrays with example data to write.
>>> a = np.arange(100*200*300, dtype=np.uint32).reshape((100, 200, 300))
>>> b = np.arange(200*300*400, dtype=np.uint32).reshape((200, 300, 400))
>>> # Initiate two asynchronous writes, to be performed concurrently.
>>> future_a = dataset[1000:1100, 2000:2200, 3000:3300].write(a)
>>> future_b = dataset[3000:3200, 4000:4300, 5000:5400].write(b)
>>> # Wait for the asynchronous writes to complete
>>> future_a.result()
>>> future_b.result()
Safe and Performant Scaling
Processing and analyzing large numerical datasets requires significant computational resources. This is typically achieved through parallelization across numerous CPU or accelerator cores spread across many machines. Therefore a fundamental goal of TensorStore has been to enable parallel processing of individual datasets that is both safe (i.e., avoids corruption or inconsistencies arising from parallel access patterns) and high performance (i.e., reading and writing to TensorStore is not a bottleneck during computation). In fact, in a test within Google’s datacenters, we found nearly linear scaling of read and write performance as the number of CPUs was increased:
 |
Read and write performance for a TensorStore dataset in zarr format residing on Google Cloud Storage (GCS) accessed concurrently using a variable number of single-core compute tasks in Google data centers. Both read and write performance scales nearly linearly with the number of compute tasks. |
Performance is achieved by implementing core operations in C++, extensive use of multithreading for operations such as encoding/decoding and network I/O, and partitioning large datasets into much smaller units through chunking to enable efficiently reading and writing subsets of the entire dataset. TensorStore also provides configurable in-memory caching (which reduces slower storage system interactions for frequently accessed data) and an asynchronous API that enables a read or write operation to continue in the background while a program completes other work.
Safety of parallel operations when many machines are accessing the same dataset is achieved through the use of optimistic concurrency, which maintains compatibility with diverse underlying storage layers (including Cloud storage platforms, such as GCS, as well as local filesystems) without significantly impacting performance. TensorStore also provides strong ACID guarantees for all individual operations executing within a single runtime.
To make distributed computing with TensorStore compatible with many existing data processing workflows, we have also integrated TensorStore with parallel computing libraries such as Apache Beam (example code) and Dask (example code).
Use Case: Language Models
An exciting recent development in ML is the emergence of more advanced language models such as PaLM. These neural networks contain hundreds of billions of parameters and exhibit some surprising capabilities in natural language understanding and generation. These models also push the limits of computational infrastructure; in particular, training a language model such as PaLM requires thousands of TPUs working in parallel.
One challenge that arises during this training process is efficiently reading and writing the model parameters. Training is distributed across many separate machines, but parameters must be regularly saved to a single object (“checkpoint”) on a permanent storage system without slowing down the overall training process. Individual training jobs must also be able to read just the specific set of parameters they are concerned with in order to avoid the overhead that would be required to load the entire set of model parameters (which could be hundreds of gigabytes).
TensorStore has already been used to address these challenges. It has been applied to manage checkpoints associated with large-scale (“multipod”) models trained with JAX (code example) and has been integrated with frameworks such as T5X (code example) and Pathways. Model parallelism is used to partition the full set of parameters, which can occupy more than a terabyte of memory, over hundreds of TPUs. Checkpoints are stored in zarr format using TensorStore, with a chunk structure chosen to allow the partition for each TPU to be read and written independently in parallel.
 |
When saving a checkpoint, each model parameter is written using TensorStore in zarr format using a chunk grid that further subdivides the grid used to partition the parameter over TPUs. The host machines write in parallel the zarr chunks for each of the partitions assigned to TPUs attached to that host. Using TensorStore's asynchronous API, training proceeds even while the data is still being written to persistent storage. When resuming from a checkpoint, each host reads only the chunks that make up the partitions assigned to that host. |
Use Case: 3D Brain Mapping
The field of synapse-resolution connectomics aims to map the wiring of animal and human brains at the detailed level of individual synaptic connections. This requires imaging the brain at extremely high resolution (nanometers) over fields of view of up to millimeters or more, which yields datasets that can span petabytes in size. In the future these datasets may extend to exabytes as scientists contemplate mapping entire mouse or primate brains. However, even current datasets pose significant challenges related to storage, manipulation, and processing; in particular, even a single brain sample may require millions of gigabytes with a coordinate system (pixel space) of hundreds of thousands pixels in each dimension.
We have used TensorStore to solve computational challenges associated with large-scale connectomic datasets. Specifically, TensorStore has managed some of the largest and most widely accessed connectomic datasets, with Google Cloud Storage as the underlying object storage system. For example, it has been applied to the human cortex “h01” dataset, which is a 3d nanometer-resolution image of human brain tissue. The raw imaging data is 1.4 petabytes (roughly 500,000 * 350,000 * 5,000 pixels large, and is further associated with additional content such as 3d segmentations and annotations that reside in the same coordinate system. The raw data is subdivided into individual chunks 128x128x16 pixels large and stored in the “Neuroglancer precomputed” format, which is optimized for web-based interactive viewing and can be easily manipulated from TensorStore.
Getting Started
To get started using the TensorStore Python API, you can install the tensorstore PyPI package using:
pip install tensorstore
Refer to the tutorials and API documentation for usage details. For other installation options and for using the C++ API, refer to installation instructions.
Acknowledgements
Thanks to Tim Blakely, Viren Jain, Yash Katariya, Jan-Matthis Luckmann, Michał Januszewski, Peter Li, Adam Roberts, Brain Williams, and Hector Yee from Google Research, and Davis Bennet, Stuart Berg, Eric Perlman, Stephen Plaza, and Juan Nunez-Iglesias from the broader scientific community for valuable feedback on the design, early testing and debugging.