Posted by Yuan Liu, PhD, Software Engineer and Peggy Bui, MD, Technical Program Manager, Google Health An estimated 1.9 billion people worldwide suffer from a skin condition at any given time, and due to a shortage of dermatologists, many cases are seen by general practitioners instead. In the United States alone, up to
37% of patients seen in the clinic have at least one skin complaint and
more than half of those patients are seen by non-dermatologists. However, studies demonstrate a significant gap in the accuracy of skin condition diagnoses between general practitioners and dermatologists, with the accuracy of general practitioners between
24% and
70%, compared to
77-
96% for dermatologists. This can lead to suboptimal referrals, delays in care, and errors in diagnosis and treatment.
Existing strategies for non-dermatologists to improve diagnostic accuracy include the use of reference textbooks, online resources, and consultation with a colleague. Machine learning tools have also been developed with the aim of helping to improve diagnostic accuracy. Previous research has largely focused on early screening of skin cancer, in particular, whether a lesion is
malignant or benign, or
whether a lesion is melanoma. However, upwards of 90% of skin problems
are not malignant, and addressing these more common conditions is also important to reduce the global burden of skin disease.
In “
A Deep Learning System for Differential Diagnosis of Skin Diseases,” we developed a deep learning system (DLS) to address the most common skin conditions seen in primary care. Our results showed that a DLS can achieve an accuracy across 26 skin conditions that is on par with U.S. board-certified dermatologists, when presented with identical information about a patient case (images and metadata). This study highlights the potential of the DLS to augment the ability of general practitioners who did not have additional specialty training to accurately diagnose skin conditions.
DLS DesignClinicians often face ambiguous cases for which there is no clear cut answer. For example, is this patient’s rash
stasis dermatitis or
cellulitis, or perhaps both superimposed? Rather than giving just one diagnosis, clinicians generate a
differential diagnosis, which is a ranked list of possible diagnoses. A differential diagnosis frames the problem so that additional workup (laboratory tests, imaging, procedures, consultations) and treatments can be systematically applied until a diagnosis is confirmed. As such, a deep learning system (DLS) that produces a ranked list of possible skin conditions for a skin complaint closely mimics how clinicians think and is key to prompt triage, diagnosis and treatment for patients.
To render this prediction, the DLS processes inputs, including one or more clinical images of the skin abnormality and up to 45 types of metadata (self-reported components of the medical history such as age, sex, symptoms, etc.). For each case, multiple images were processed using the
Inception-v4 neural network architecture and combined with feature-transformed metadata, for use in the classification layer. In our study, we developed and evaluated the DLS with 17,777 de-identified cases that were primarily referred from primary care clinics to a
teledermatology service. Data from 2010-2017 were used for training and data from 2017-2018 for evaluation. During model training, the DLS leveraged over 50,000 differential diagnoses provided by over 40 dermatologists.
To evaluate the DLS’s accuracy, we compared it to a rigorous reference standard based on the diagnoses from three U.S. board-certified dermatologists. In total, dermatologists provided differential diagnoses for 3,756 cases (“Validation set A”), and these diagnoses were aggregated via a voting process to derive the ground truth labels. The DLS’s ranked list of skin conditions was compared with this dermatologist-derived differential diagnosis, achieving 71% and 93% top-1 and top-3 accuracies, respectively.
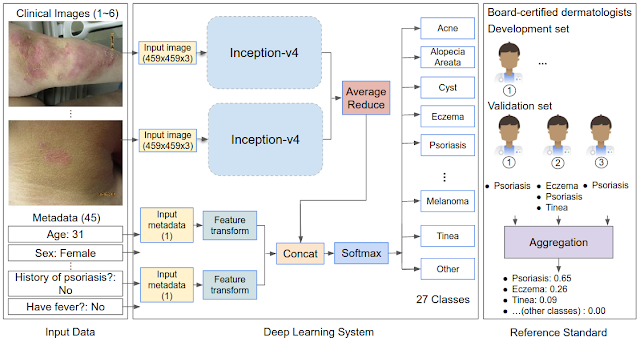 |
Schematic of the DLS and how the reference standard (ground truth) was derived via the voting of three board-certified dermatologists for each case in the validation set. |
Comparison to Professional EvaluationsIn this study, we also compared the accuracy of the DLS to that of three categories of clinicians on a subset of the validation A dataset (“Validation set B”): dermatologists, primary care physicians (PCPs), and nurse practitioners (NPs) — all chosen randomly and representing a range of experience, training, and diagnostic accuracy. Because typical differential diagnoses provided by clinicians only contain up to three diagnoses, we compared only the top three predictions by the DLS with the clinicians. The DLS achieved a top-3 diagnostic accuracy of 90% on the validation B dataset, which was comparable to dermatologists and substantially higher than primary care physicians (PCPs) and nurse practitioners (NPs)—75%, 60%, and 55%, respectively, for the 6 clinicians in each group. This high top-3 accuracy suggests that the DLS may help prompt clinicians (including dermatologists) to consider possibilities that were not originally in their differential diagnoses, thus improving diagnostic accuracy and condition management.
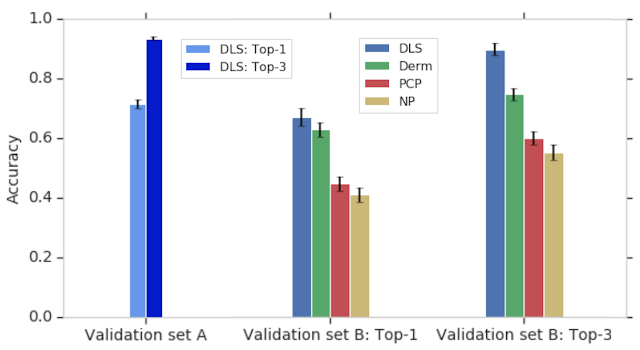 |
The DLS’s leading (top-1) differential diagnosis is substantially higher than PCPs and NPs, and on par with dermatologists. This accuracy increases substantially when we look at the DLS’s top-3 accuracy, suggesting that in the majority of cases the DLS’s ranked list of diagnoses contains the correct ground truth answer for the case. |
Assessing Demographic PerformanceSkin type, in particular, is highly relevant to dermatology, where visual assessment of the skin itself is crucial to diagnosis. To evaluate potential bias towards skin type, we examined DLS performance based on the
Fitzpatrick skin type, which is a scale that ranges from Type I (“pale white, always burns, never tans”) to Type VI (“darkest brown, never burns”). To ensure sufficient numbers of cases on which to draw convincing conclusions, we focused on skin types that represented at least 5% of the data — Fitzpatrick skin types II through IV. On these categories, the DLS’s accuracy was similar, with a top-1 accuracy ranging from 69-72%, and the top-3 accuracy from 91-94%. Encouragingly, the DLS also remained accurate in patient subgroups for which significant numbers (at least 5%) were present in the dataset based on other self-reported demographic information: age, sex, and race/ethnicities. As further qualitative analysis, we assessed via saliency (explanation) techniques that the DLS was reassuringly “focusing” on the abnormalities instead of on skin tone.
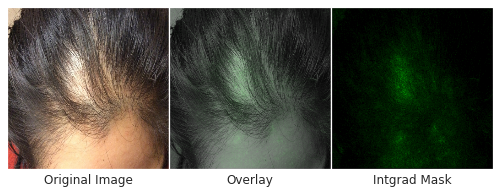 |
Left: An example of a case with hair loss that was challenging for non-specialists to arrive at the specific diagnosis, which is necessary for determining appropriate treatment. Right: An image with regions highlighted in green showing the areas that the DLS identified as important and used to make its prediction. Center: The combined image, which indicates that the DLS mostly focused on the area with hair loss to make this prediction, instead of on forehead skin color, for example, which may indicate potential bias. |
Incorporating Multiple Data TypesWe also studied the effect of different types of input data on the DLS performance. Much like how having images from several angles can help a teledermatologist more accurately diagnose a skin condition, the accuracy of the DLS improves with increasing number of images. If metadata (e.g., the medical history) is missing, the model does not perform as well. This accuracy gap, which may occur in scenarios where no medical history is available, can be partially mitigated by training the DLS with only images. Nevertheless, this data suggests that providing the answers to a few questions about the skin condition can substantially improve the DLS accuracy.
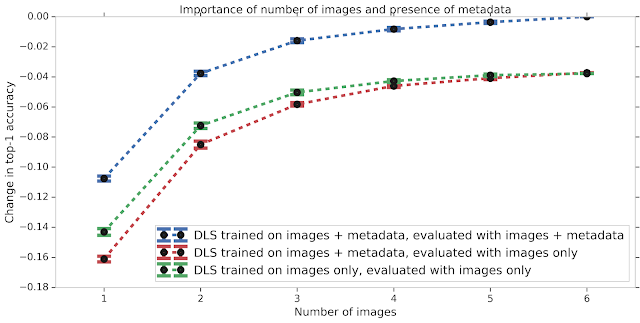 |
The DLS performance improves when more images (blue line) or metadata (blue compared with red line) are present. In the absence of metadata as input, training a separate DLS using images alone leads to a marginal improvement compared to the current DLS (green line). |
Future Work and ApplicationsThough these results are very promising, much work remains ahead. First, as reflective of real-world practice, the relative rarity of skin cancer such as melanoma in our dataset hindered our ability to train an accurate system to detect cancer. Related to this, the skin cancer labels in our dataset were not biopsy-proven, limiting the quality of the ground truth in this regard. Second, while our dataset did contain a variety of Fitzpatrick skin types, some skin types were too rare in this dataset to allow meaningful training or analysis. Finally, the validation dataset was from one teledermatology service. Though 17 primary care locations across two states were included, additional validation on cases from a wider geographical region will be critical. We believe these limitations can be addressed by including more cases of biopsy-proven skin cancers in the training and validation sets, and including cases representative of additional Fitzpatrick skin types and from other clinical centers.
The success of deep learning to inform the differential diagnosis of skin disease is highly encouraging of such a tool’s potential to assist clinicians. For example, such a DLS could help triage cases to guide prioritization for clinical care or could help non-dermatologists initiate dermatologic care more accurately and potentially improve access. Though significant work remains, we are excited for future efforts in examining the usefulness of such a system for clinicians. For research collaboration inquiries, please contact
[email protected].
AcknowledgementsThis work involved the efforts of a multidisciplinary team of software engineers, researchers, clinicians and cross functional contributors. Key contributors to this project include Yuan Liu, Ayush Jain, Clara Eng, David H. Way, Kang Lee, Peggy Bui, Kimberly Kanada, Guilherme de Oliveira Marinho, Jessica Gallegos, Sara Gabriele, Vishakha Gupta, Nalini Singh, Vivek Natarajan, Rainer Hofmann-Wellenhof, Greg S. Corrado, Lily H. Peng, Dale R. Webster, Dennis Ai, Susan Huang, Yun Liu, R. Carter Dunn and David Coz. The authors would like to acknowledge William Chen, Jessica Yoshimi, Xiang Ji and Quang Duong for software infrastructure support for data collection. Thanks also go to Genevieve Foti, Ken Su, T Saensuksopa, Devon Wang, Yi Gao and Linh Tran. Last but not least, this work would not have been possible without the participation of the dermatologists, primary care physicians, nurse practitioners who reviewed cases for this study, Sabina Bis who helped to establish the skin condition mapping and Amy Paller who provided feedback on the manuscript.