Posted by Matthew Burgess and Natasha Noy, Google AIEarlier this month we launched
Google Dataset Search, a tool designed to make it easier for researchers to discover datasets that can help with their work. What we colloquially call "Google Scholar for data,” Google Dataset Search is a search engine across metadata for millions of datasets in thousands of repositories across the Web. In this post, we go into some detail of how Dataset Search is built, outlining what we believe will help develop an open data ecosystem, and we also address the question that we received frequently since the Dataset Search
launch, "
Why is my dataset not showing up in Google Dataset Search?”
An OverviewAt a very high level, Google Data Search relies on dataset providers, big and small,
adding structured metadata on their sites using the open
schema.org/Dataset standard. The metadata specifies the salient properties of each dataset: its name and description, spatial and temporal coverage, provenance information, and so on. Dataset Search uses this metadata, links it with other resources that are available at Google (more on this below!), and builds an index of this enriched corpus of metadata. Once we built the index, we can start answering user queries — and figuring out which results best correspond to the query.
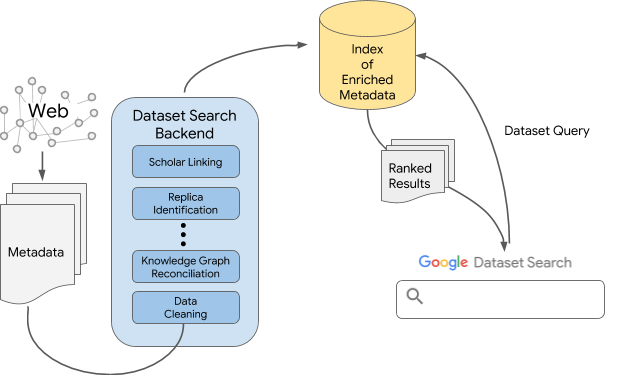 |
An overview of the technology behind Google Dataset Search |
Using Structured Metadata from Data ProvidersWhen Google's search engine processes a Web page with schema.org/Dataset mark-up, it understands that there is dataset metadata there and processes that structured metadata to create "records" describing each annotated dataset on a page. The use of schema.org allows developers to embed this structured information into HTML, without affecting the appearance of the page while making the semantics of the information visible to all search engines.
However, no matter how precise schema.org definitions or
guidelines are, some metadata will inevitably be incomplete, wrong, or entirely missing. Furthermore, distinctions between some fields can be vague: is the dataset repository a publisher or a provider of a dataset? How do we distinguish between citations to a scientific paper that describes the creation of the dataset vs. papers describing its use? Indeed, many of these questions often generate active
scholarly discussions.
Despite these variations, Dataset Search must provide a uniform and predictable user experience on the front end. Therefore, in some cases we substitute a more general field name (e.g., “
provided by”) to display the values coming from multiple other fields (e.g., “
publisher”, “
creator”, etc.). In other cases, we are not able to use some of the fields at all: if a specific field is being misinterpreted in many different ways by dataset providers, we bypass that field for now and work with the community to clarify the guidelines. In each decision, we had one specific question that helped us in difficult cases "What will help data discovery the most?" This focus on the task that we were addressing made some of the problems easier than they seemed at first.
Connecting Replicas of DatasetsIt is very common for a dataset, in particular a popular one, to be present in more than one repository. We use a variety of signals to determine when two datasets are replicas of each other. For example, schema.org has a way to specify the connection explicitly, through
schema.org/sameAs, which is the best way to link different replicas together and to point to the canonical source of a dataset. Other signals include two datasets descriptions pointing to the same canonical page, having the same
Digital Object Identifier (DOI), sharing links for downloading the dataset, or having a large overlap in other metadata fields. None of these signals are perfect in isolation, therefore we combine them to get the strongest possible indication of when two datasets are the same.
Reconciling to the Google Knowledge Graph Google's
Knowledge Graph is a powerful platform that describes and links information about many entities, including the ones that appear in dataset metadata: organizations providing datasets, locations for spatial coverage of the data, funding agencies, and so on. Therefore, we try to reconcile information mentioned in the metadata fields with the items in the Knowledge Graph. We can do this reconciliation with good precision for two main reasons. First, we know the types of items in the Knowledge Graph and the types of entities that we expect in the metadata fields. Therefore, we can limit the types of entities from the Knowledge Graph that we match with values for a particular metadata field. For example, a provider of a dataset should match with an organization entity in the Knowledge Graph and not with, say, a location. Second, the context of the Web page itself helps reduce the number of choices, which is particularly useful for distinguishing between organizations that share the same acronym. For example, the acronym CAMRA can stand for “Chilbolton Advanced Meteorological Radar” or “Campaign for Real Ale”. If we use terms from the Web page, we can then more easily determine that CAMRA is in fact the Chilbolton Radar when we see terms such as “
clouds”, “
vapor”, and “
water” on the page.
This type of reconciliation opens up lots of possibilities to improve the search experience for users. For instance, Dataset Search can localize results by showing reconciled values of metadata in the same language as the rest of the page. Additionally, it can rely on synonyms, correct misspellings, expand acronyms, or use other relations in the Knowledge Graph for query expansion.
Linking to other Google Resources Google has many other data resources that are useful in augmenting the dataset metadata, such as
Google Scholar. Knowing which datasets are referenced and cited in publications serves at least two purposes:
- It provides a valuable signal about the importance and prominence of a dataset.
- It gives dataset authors an easy place to see citations to their data and to get credit.
Indeed, we hope that highlighting publications that use the data will lead to a more healthy ecosystem of data citation. For the moment, our links to Google scholar are very approximate as we lack a good model on how people cite data. We try to go beyond DOIs to give somewhat better coverage, but the number of articles citing a dataset ends up being approximate. We hope to make more progress in this area in order to get a higher level of precision.
Search and Ranking of ResultsWhen a user issues a query, we search through the corpus of datasets, in a way not unlike Google Search works over Web pages. Just like with any search, we need to determine whether a document is relevant for the query and then rank the relevant documents. Because there are no large-scale studies on how users search for datasets, as a first approximation, we rely on Google Web ranking. However, ranking datasets is different from ranking Web pages, and we add some additional signals that take into account the metadata quality, citations, and so on. As Dataset Search gets used more by our users and we understand better how users search for datasets, we hope that ranking will improve significantly.
A Better Open Data EcosystemWe built Dataset Search in an attempt to create a tool that will positively impact the discoverability of data. The decision to rely on open standards (
schema.org,
W3C DCAT,
JSON-LD, etc.) for markup is intentional, as Dataset Search can only be as good as the open-data ecosystem that it supports. As such, Google Dataset Search aims to support a strong open data ecosystem by encouraging:
- Widespread adoption of open metadata formats to describe published data.
- Further development of open metadata formats to describe more types of data and in more detail.
- The culture of citing data the way we cite research publications, giving those who create and publish the data the credit that they deserve.
- The development of tools that leverage this metadata to enable more discovery or better use of data.
The increased adoption of open metadata standards in conjunction with the continued development of Dataset Search (and, hopefully, other tools) should foster a healthier open data ecosystem where data is a first-class citizen of research.
So, Where is Your Dataset?It is probably clear by now that Dataset Search is only as good as the metadata that exists on the Web pages for datasets. The most common answer to the question of why a specific dataset does not show up in our results is that the Web page for that dataset does not have any markup. Just pop that page into the
Structured Data Testing Tool and you will see whether the markup is there. If you don't see any markup there, and you own the page, you can
add it and if you don't own the page, you can ask the page owners to do it, which will make their page more easily discoverable by everyone.
We hope that the community finds
Dataset Search useful, users make serendipitous discoveries and save time and scientists and journalists spend less time searching for data and more time using it.
AcknowledgementsWe would like to thank Xiaomeng Ban, Dan Brickley, Lee Butler, Thomas Chen, Corinna Cortes, Kevin Espinoza, Archana Jain, Mike Jones, Kishore Papineni, Chris Sater, Gokhan Turhan, Shubin Zhao and Andi Vajda for their work on the project and all our partners, collaborators, and early adopters for their help.