As the publishing world continues to face new challenges amidst the shift to digital, news media and publishers are tasked with unlocking new opportunities. With online news consumption continuing to grow, it’s crucial that publishers take advantage of new technologies to sustain and grow their business. Machine learning yields tremendous value for media and can help them tackle the hardest problems: engaging readers, increasing profits, and making newsrooms more efficient. Google has a suite of machine learning tools and services that are easy to use—here are a few ways they can help newsrooms and reporters do their jobs
1. Improve your newsroom's efficiency
Editors want to make their stories appealing and to stand out so that people will read them. So finding just the right photograph or video can be key in bringing a story to life. But with ever-pressing deadlines, there’s often not enough time to find that perfect image. This is where Google Cloud Vision and Video Intelligence can simplify the process by tagging images and videos based on the content inside the actual image. This metadata can then be used to make it easier and quicker to find the right visual.
2. Better understand your audience
News publishers use analytics tools to grow their audiences, and understand what that audience is reading and how they’re discovering content. Google Cloud Natural Language uses machine learning to understand what your content is about, independent of a website’s section and subsection structure (i.e. Sports, Local, etc.) Today, Cloud Natural Language announced a new content classifier and entity sentiment that digs into the detail of what a story is actually about. For example, an article about a high-tech stadium for the Golden State Warriors may be classified under the “technology” section of a paper, when its content should fall under “technology” and “sports.” This section-independent tagging can increase readership by driving smarter article recommendations and provides better data around trending topics. Naveed Ahmad, Senior Director of Data at Hearst has emphasized that precision and speed are critical to engaging readers: “Google Cloud Natural Language is unmatched in its accuracy for content classification. At Hearst, we publish several thousand articles a day across 30+ properties and, with natural language processing, we're able to quickly gain insight into what content is being published and how it resonates with our audiences."
3. Engage with new audiences
As publications expand their reach into more countries, they have to write for multiple audiences in different languages and many cannot afford multi-language desks. Google Cloud Translation makes translating for different audiences easier by providing a simple interface to translate content into more than 100 languages. Vice launched GoogleFish earlier this year to help editors quickly translate existing Vice articles into the language of their market. Once text was auto-translated, an editor could then push the translation to a local editor to ensure tone and local slang were accurate. Early translation results are very positive and Vice is also uncovering new insights around global content sharing they could not previously identify.
DB Corp, India’s largest newspaper group, publishes 62 editions in four languages and sells about 6 million newspaper copies per day. To address its growing customers and its diverse readership, reporters use Google Cloud Translation to capture and document interviews and source material for articles, with accuracy rates of 95 percent for Hindi alone.
4. Monetize your audience
So far we’ve primarily outlined ways to improve content creation and engagement with readers, however monetization is a critical piece for all publishers. Using Cloud Datalab, publishers can identify new subscription opportunities and offerings. The metadata collected from image, video, and content tagging creates an invaluable dataset to advertisers, such as audiences interested in local events or personal finance, or those who watch videos about cars or travel. The Washington Post has seen success with their in-house solution through the ability to target native ads to likely interested readers. Lastly, improved content recommendation drives consumption, ultimately improving the bottom line.
5. Experiment with new formats
The ability to share news quickly and efficiently is a major concern for newsrooms across the world. However today more than ever, readers are reading the news in different ways across different platforms and the “one format fits all” method is not always best. TensorFlow’s “summary.text” feature can help publishers quickly experiment with creating short form content from longer stories. This helps them quickly test the best way to share their content across different platforms. Reddit recently launched a similar “tl;dr bot” that summarizes long posts into digestible snippets.
6. Keep your content safe for everyone
The comments section can be a place of both fruitful discussion as well as toxicity. Users who comment are frequently the most highly engaged on the site overall, and while publishers want to keep sharing open, it can frequently spiral out of control into offensive speech and bad language. Jigsaw’s Perspective is an API that uses machine learning to spot harmful comments which can be flagged for moderators. Publishers like the New York Times have leveraged Perspective's technology to improve the way all readers engage with comments. By making the task of moderating conversations at scale easier, this frees up valuable time for editors and improves online discussion.
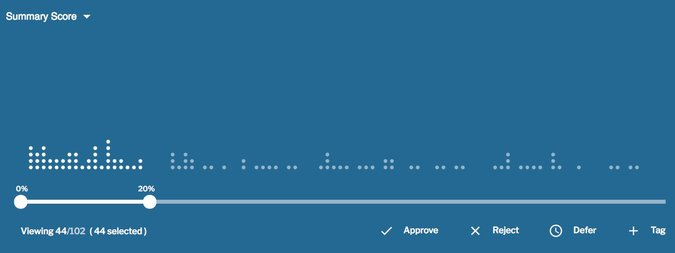
From the printing press to machine learning, technology continues to spur new opportunities for publishers to reach more people, create engaging content and operate efficiently. We're only beginning to scratch the surface of what machine learning can do for publishers. Keep tabs on The Keyword for the latest developments.