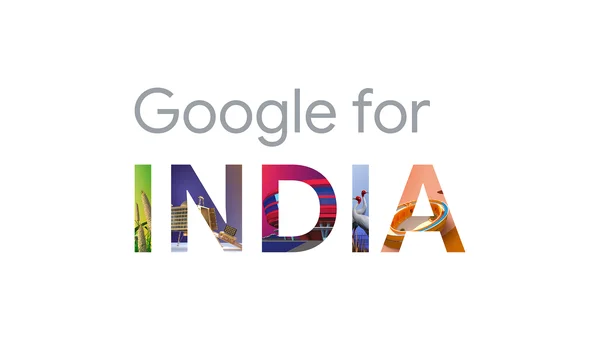
5 things we announced at Google for India 2023
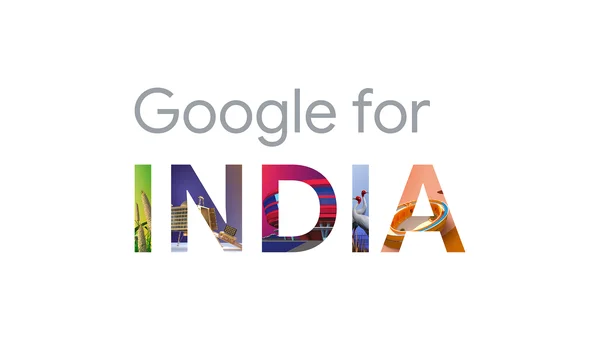
Welcome to the latest edition of “My Path to Google,” where we talk to Googlers, interns and alumni about how they got to Google, what their roles are like and even some tips on how to prepare for interviews.
Today’s post is all about Sameer Sethi, a lead technical program manager on our Responsible AI team.
Can you tell us a little bit about yourself?
I was born and raised in a Punjabi family in Delhi, India. Growing up, my parents were my role models and the biggest pillars in my life. My passions are trying different foods and drinks and exploring the world with my wife, who I met while traveling.
Walk us through your path to Google.
After completing my Bachelor’s of Engineering in Dublin, I worked as a software engineer at various telecom companies in Ireland. Afterwards, I moved back to India to help take care of my father and ended up opening an Indo-European bakery. While I enjoyed running a small business (especially one dedicated to my love of food), I still wanted to find an outlet for my engineering skills and use them to create a positive impact. So I got my master’s degree in engineering and ventured back into the tech world as a product manager.
I partnered with Google on a project during that time, and having seen my work firsthand, my Google colleagues encouraged me to apply for an open position in Google Fiber. The role tapped into my passion for data engineering, and I could make a difference by helping to provide fast, reliable and fairly priced internet access to everyone. So I applied and got the job.
What’s your role at Google now?
Now, I’m a lead technical program manager on Google’s Responsible AI team. I work with researchers, ethicists and machine learning practitioners to help Google build responsible AI products guided by our AI Principles and the societal impacts of our work.
What do you wish you’d known when you started the interview process?
I read about Google’s interview process online and got the impression that it would be a nerve-racking experience. To my surprise, however, it was quite the opposite. While the interviews definitely required a lot of preparation, all of the interviewers were very friendly, and the process is designed to make you feel comfortable and help you do your best. Looking back, I would have been much less nervous knowing that the interviewers were friendly and there to help me succeed.
What resources would you recommend to prepare for a Google interview?
I’d suggest reading about the hiring process and checking out the Life at Google YouTube channel to hear directly from Googlers about what it’s like to work here, and how to best prepare to apply or interview. And remember, your recruiter is your best ally so take their recommendations. They know the Google hiring process inside out.
Any tips you’d like to share with aspiring Googlers?
I’ll second what many other Googlers have said: Always strive for your best, prepare well and understand that the interviewers are there to help you succeed. Don’t lose hope, and never stop dreaming.
This year, the Hindu chapter of Google's Inter Belief Network (IBN) employee resource group is honoring Hindu Heritage Month and the many important festivals happening this month, including Navaratri (Sept. 26 - Oct. 5), Dussehra (Oct. 5) and Diwali (Oct. 24).
To help bring more awareness to key moments like Diwali, Google Registry partnered withCoHNA, a coalition representing the Hindu community of North America, earlier this year to launch Diwali.day — a secure domain to learn more about the history and traditions surrounding Diwali.
I sat down with fellow members of the IBN Hindu chapter to learn a bit more about their traditions, and how they are celebrating the festive season.
The widespread availability of mobile phones has enabled non-profits to deliver critical health information to their beneficiaries in a timely manner. While advanced applications on smartphones allow for richer multimedia content and two-way communication between beneficiaries and health coaches, simpler text and voice messaging services can be effective in disseminating information to large communities, particularly those that are underserved with limited access to information and smartphones. ARMMAN1, one non-profit doing just this, is based in India with the mission of improving maternal and child health outcomes in underserved communities.
![]() |
Overview of ARMMAN |
One of the programs run by them is mMitra, which employs automated voice messaging to deliver timely preventive care information to expecting and new mothers during pregnancy and until one year after birth. These messages are tailored according to the gestational age of the beneficiary. Regular listenership to these messages has been shown to have a high correlation with improved behavioral and health outcomes, such as a 17% increase in infants with tripled birth weight at end of year and a 36% increase in women knowing the importance of taking iron tablets.
However, a key challenge ARMMAN faced was that about 40% of women gradually stopped engaging with the program. While it’s possible to mitigate this with live service calls to women to explain the advantage of listening to the messages, it is infeasible to call all the low listeners in the program because of limited support staff — this highlights the importance of effectively prioritizing who receives such service calls.
In “Field Study in Deploying Restless Multi-Armed Bandits: Assisting Non-Profits in Improving Maternal and Child Health”, published in AAAI 2022, we describe an ML-based solution that uses historical data from the NGO to predict which beneficiaries will benefit most from service calls. We address the challenges that come with a large-scale real world deployment of such a system and show the usefulness of deploying this model in a real study involving over 23,000 participants. The model showed an increase in listenership of 30% compared to the current standard of care group.
Background
We model this resource optimization problem using restless multi-armed bandits (RMABs), which have been well studied for application to such problems in a myriad of domains, including healthcare. An RMAB consists of n arms where each arm (representing a beneficiary) is associated with a two-state Markov decision process (MDP). Each MDP is modeled as a two-state (good or bad state, where the good state corresponds to high listenership in the previous week), two-action (corresponding to whether the beneficiary was chosen to receive a service call or not) problem. Further, each MDP has an associated reward function (i.e., the reward accumulated at a given state and action) and a transition function indicating the probability of moving from one state to the next under a given action, under the Markov condition that the next state depends only on the previous state and the action taken on that arm in that time step. The term restless indicates that all arms can change state irrespective of the action.
![]() |
State of a beneficiary may transition from good (high engagement) to bad (low engagement) with example passive and active transition probabilities shown in the transition matrix. |
Model Development
Finally, the RMAB problem is modeled such that at any time step, given n total arms, which k arms should be acted on (i.e., chosen to receive a service call), to maximize reward (engagement with the program).
The probability of transitioning from one state to another with (active probability) or without (passive probability) receiving a service call are therefore the underlying model parameters that are critical to solving the above optimization. To estimate these parameters, we use the demographic data of the beneficiaries collected at time of enrolment by the NGO, such as age, income, education, number of children, etc., as well as past listenership data, all in-line with the NGO’s data privacy standards (more below).
However, the limited volume of service calls limits the data corresponding to receiving a service call. To mitigate this, we use clustering techniques to learn from the collective observations of beneficiaries within a cluster and enable overcoming the challenge of limited samples per individual beneficiary.
In particular, we perform clustering on listenership behaviors, and then compute a mapping from the demographic features to each cluster.
![]() |
Clustering on past listenership data reveals clusters with beneficiaries that behave similarly. We then infer a mapping from demographic features to clusters. |
This mapping is useful because when a new beneficiary is enrolled, we only have access to their demographic information and have no knowledge of their listenership patterns, since they haven’t had a chance to listen yet. Using the mapping, we can infer transition probabilities for any new beneficiary that enrolls into the system.
We used several qualitative and quantitative metrics to infer the optimal set of of clusters and explored different combinations of training data (demographic features only, features plus passive probabilities, features plus all probabilities, passive probabilities only) to achieve the most meaningful clusters, that are representative of the underlying data distribution and have a low variance in individual cluster sizes.
Clustering has the added advantage of reducing computational cost for resource-limited NGOs, as the optimization needs to be solved at a cluster level rather than an individual level. Finally, solving RMAB’s is known to be P-space hard, so we choose to solve the optimization using the popular Whittle index approach, which ultimately provides a ranking of beneficiaries based on their likely benefit of receiving a service call.
Results
We evaluated the model in a real world study consisting of approximately 23,000 beneficiaries who were divided into three groups: the current standard of care (CSOC) group, the "round robin" (RR) group, and the RMAB group. The beneficiaries in the CSOC group follow the original standard of care, where there are no NGO initiated service calls. The RR group represents the scenario where the NGO often conducts service calls using some systematic set order — the idea here is to have an easily executable policy that services enough of a cross-section of beneficiaries and can be scaled up or down per week based on available resources (this is the approach used by the NGO in this particular case, but the approach may vary for different NGOs). The RMAB group receives service calls as predicted by the RMAB model. All the beneficiaries across the three groups continue to receive the automated voice messages independent of the service calls.
At the end of seven weeks, RMAB-based service calls resulted in the highest (and statistically significant) reduction in cumulative engagement drops (32%) compared to the CSOC group.
![]() |
The plot shows cumulative engagement drops prevented compared to the control group. |
RMAB vs CSOC | RR vs CSOC | RMAB vs RR | |
% reduction in cumulative engagement drops | 32.0% | 5.2% | 28.3% |
p-value | 0.044 | 0.740 | 0.098 |
Ethical Considerations
An ethics board at the NGO reviewed the study. We took significant measures to ensure participant consent is understood and recorded in a language of the community's choice at each stage of the program. Data stewardship resides in the hands of the NGO, and only the NGO is allowed to share data. The code will soon be available publicly. The pipeline only uses anonymized data and no personally identifiable information (PII) is made available to the models. Sensitive data, such as caste, religion, etc., are not collected by ARMMAN for mMitra. Therefore, in pursuit of ensuring fairness of the model, we worked with public health and field experts to ensure other indicators of socioeconomic status were measured and adequately evaluated as shown below.
![]() |
Distribution of highest education received (top) and monthly family income in Indian Rupees (bottom) across a cohort that received service calls compared to the whole population. |
The proportion of beneficiaries that received a live service call within each income bracket reasonably matches the proportion in the overall population. However, differences are observed in lower income categories, where the RMAB model favors beneficiaries with lower income and beneficiaries with no formal education. Lastly, domain experts at ARMMAN have been deeply involved in the development and testing of this system and have provided continuous input and oversight in data interpretation, data consumption, and model design.
Conclusions
After thorough testing, the NGO has currently deployed this system for scheduling of service calls on a weekly basis. We are hopeful that this will pave the way for more deployments of ML algorithms for social impact in partnerships with non-profits in service of populations that have so far benefited less from ML. This work was also featured in Google for India 2021.
Acknowledgements
This work is part of our AI for Social Good efforts and was led by Google Research, India. Thanks to all our collaborators at ARMMAN, Google Research India, Google.org, and University Relations: Aparna Hegde, Neha Madhiwalla, Suresh Chaudhary, Aditya Mate, Lovish Madaan, Shresth Verma, Gargi Singh, Divy Thakkar.
1ARMMAN runs multiple programs to provide preventive care information to women through pregnancy and infancy enabling them to seek care, as well as programs to train and support health workers for timely detection and management of high-risk conditions. ↩