[Editor's note: This is one of many posts on enterprise features enabled by Kubernetes Engine 1.10. For the full coverage, follow along here.]
Over the past few weeks, we’ve made some exciting announcements around Google Kubernetes Engine, starting with the general availability of Kubernetes 1.10 in the service. This latest version has new features that will really help enterprise use cases such as support for Shared Virtual Private Cloud (VPC) and Regional Clusters for high availability and reliability.
Building on that momentum, we are excited to announce the ability to create VPC-native clusters in Kubernetes Engine. A VPC-native cluster uses Alias IP routing built into the VPC network, resulting in a more scalable, secure and simple system that is suited for demanding enterprise deployments and use cases.
VPC-native clusters using Alias IP
VPC-native clusters rely on Alias IP which provides integrated VPC support for container networking. Without Alias IP, Kubernetes Engine uses Routes for Pod networking, which requires the Kubernetes control plane to maintain static routes to each Node. By using Alias IP, the VPC control panel automatically manages routing setup for Pods. In addition to this automatic management, native integration of container networking into the VPC fabric improves scalability and integration between Kubernetes and other VPC features.
Alias IP has been available on Google Cloud Platform (GCP) for Google Compute Engine instances for some time. Extending this functionality to Kubernetes Engine provides the following benefits:
- Scale enhancements - VPC-native clusters no longer carry the burden of Routes and can scale to more nodes. VPC-native clusters will not be subject to Route quotas and limits, allowing you to seamlessly increase your Cluster size.
- Hybrid connectivity - Alias IP subnets can be advertised by the Cloud Router over Cloud VPN or Cloud Interconnect, allowing you to connect your hybrid on-premises deployments with your Kubernetes Engine cluster. In addition, Alias IP advertisements with Cloud Router gives you granular control over which subnetworks and secondary range(s) are published to peer routers.
- Better VPC integration - Alias IP provides Kubernetes Engine Pods with direct access to Google services like Google Cloud Storage, BigQuery and any other services served from the googleapis.com domain, without the overhead of a NAT proxy. Alias IP also enables enhanced VPC features such as Shared VPC.
- Security checks - Alias IP allows you to enable anti-spoofing checks for the Nodes in your cluster. These anti-spoofing checks are provisioned on instances by default to ensure that traffic is not sent from arbitrary source IPs. Since Alias IP ranges in VPC-native clusters are known to the VPC network, they pass anti-spoofing checks by gidefault.
- IP address management - VPC-native clusters integrate directly into your VPC IP address management system, preventing potential double allocation of your VPC IP space. Route-based clusters required manually blocking off the set of IPs assigned to your Cluster. VPC-native clusters provide two modes of allocating IPs, providing a full spectrum of control to the user. In the default method, Kubernetes Engine auto-selects and assigns secondary ranges for Pods and Services ranges. And if you need tight control over subnet assignments, you can create a custom subnet and secondary ranges and use it for Node, Pods and Service IPs. With Alias IP, GCP ensures that the Pod IP addresses cannot conflict with IP addresses on other resources.
“VPC-native clusters, using Alias IPs, in Google Kubernetes Engine allowed us to run our bandwidth-hungry applications on Kubernetes without any of the performance degradation that we had seen when using overlay networks."Try it out today!
- Daniel Nelson, Director of Engineering, Vungle
Create VPC-native clusters in Kubernetes Engine to get the ease of access and scale enterprise workloads require. Also, don’t forget to sign up for our upcoming webinar, 3 reasons why you should run your enterprise workloads on Google Kubernetes Engine.
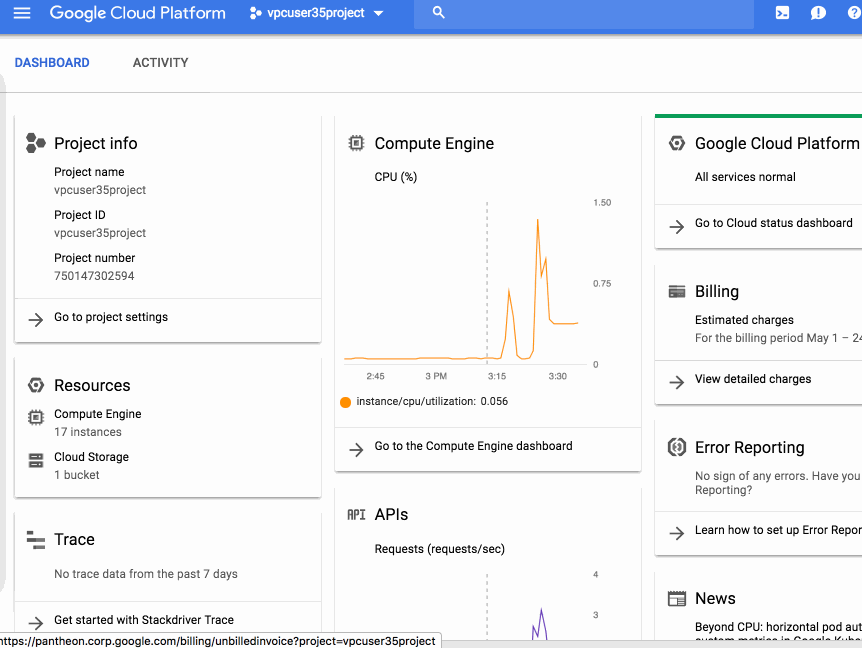