Five years ago, we open-sourced TensorFlow, our machine learning framework for research and production. Our goal was to expand access to state-of-the-art machine learning tools so anyone could use them.
Since then, TensorFlow has become the most popular machine learning library in the world, with over 160 million downloads. Seeing so many people use TensorFlow is an incredible and humbling experience, and we’re thankful for the thousands of people outside of Google who have contributed code, created educational content and organized developer events around the world to support TensorFlow and the growing machine learning community.
To celebrate five years of TensorFlow, we’d like to point out a few interactive demos you can try from your browser with a single click, as well as some tutorials that can help you create your own projects. If you’re new to TensorFlow, these are a great way to get a feel for what it can do. And if you like what you see and want to dive a bit deeper, check out the TensorFlow Blog.
Try out some interactive demos powered by machine learning
TensorFlow supports multiple programming languages and environments. Let’s start with a quick tour of JavaScript, and three interactive demos you can try with a click.
TensorFlow.js enables you to write and run machine learning models entirely in the browser. This has important applications for privacy preserving applications (no data needs to be sent to a server), and for interactive machine learning programs.
One great example of this is this iris landmark-tracking program which supports hands-free interfaces and assistive technologies; you can try the model yourself in your browser (be patient—it may take a few moments to load!).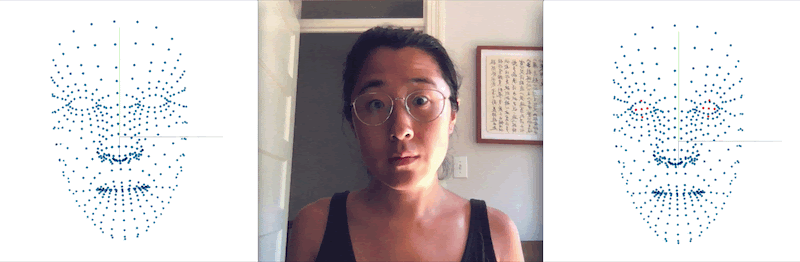
Similarly to eye-tracking, you can also use TensorFlow.js to track hand motions.
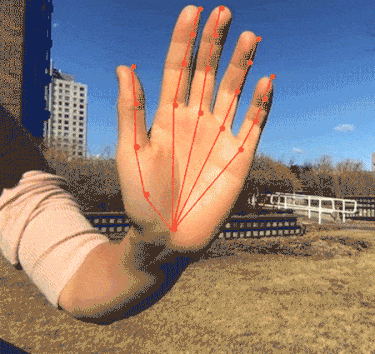
You only need a webcam for both of these demos, and no data leaves your machine.
Train your own model, no coding necessary
You can train your own model (with no coding required) using the Teachable Machine. It’s a fast, fun, and easy way to create a machine learning model right in your browser. For instance, you could teach a model to recognize images, or sounds that you record using your microphone.

Go deeper with tutorials
TensorFlow includes a powerful Python library. To get started using it, here are some tutorials for beginners and experts alike. These tutorials (which contain complete, end-to-end code) span topics from machine learning fundamentals, to computer vision and machine translation—and even show you how to generate artwork with machine learning.
Image CC-BY by Virginia McMillan.
Bring TensorFlow to mobile apps
TensorFlow Lite enables you to build machine learning-powered apps on mobile and small embedded devices. A group of engineering students in India used TensorFlow Lite to develop an Android app that provides local air quality information using a smartphone camera.
You can go even smaller, too: TensorFlow Lite Micro lets you run machine learning models on microcontrollers (tiny computers that can fit in the palm of your hand).
Understand how to build responsibly
As billions of people around the world continue to use products and services with machine learning at their core, it’s become increasingly important to design and deploy these systems responsibly. TensorFlow includes a large set of tools and best practices for Responsible AI, including the What-If Tool which tests how machine learning models will work for different people in hypothetical situations.And there’s much more you can do as well. TensorFlow includes a complete set of tools to power production ML systems, and even supports the latest research in Quantum computing.
This is only the beginning, and we’re excited to see what the next five years bring. To learn more about TensorFlow, check out tensorflow.org, read the blog, follow us on social or subscribe to our YouTube Channel.