Editor’s Note: Do you ever feel like a fish out of water? Try being a tech novice and talking to an engineer at a place like Google. Ask a Techspert is a series on the Keyword asking Googler experts to explain complicated technology for the rest of us. This isn’t meant to be comprehensive, but just enough to make you sound smart at a dinner party.
A few years ago, I learned that a translation from Finnish to English using Google Translate led to an unexpected outcome. The sentence “hän on lentäjä” became “he is a pilot” in English, even though “hän” is a gender-neutral word in Finnish. Why did Translate assume it was “he” as the default?
As I started looking into it, I became aware that just like humans, machines are affected by society’s biases. The machine learning model for Translate relied on training data, which consisted of the input from hundreds of millions of already-translated examples from the web. “He” was more associated with some professions than “she” was, and vice versa.
Now, Google provides options for both feminine and masculine translations when adapting gender-neutral words in several languages, and there’s a continued effort to roll it out more broadly. But it’s still a good example of how machine learning can reflect the biases we see all around us. Thankfully, there are teams at Google dedicated to finding human-centered solutions to making technology inclusive for everyone. I sat down with Been Kim, a Google researcher working on the People + AI Research (PAIR) team, who devotes her time to making sure artificial intelligence puts people, not machines, at its center, and helping others understand the full spectrum of human interaction with machine intelligence. We talked about how you make machine learning models easy to interpret and understand, and why it’s important for everybody to have a basic idea of how the technology works.
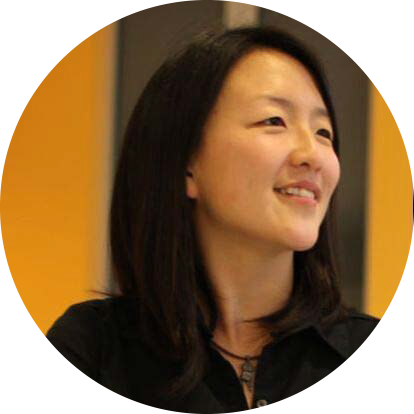
Why is this field of work so important?
Machine learning is such a powerful tool, and because of that, you want to make sure you’re using it responsibly. Let’s take an electric machine saw as an example. It’s a super powerful tool, but you need to learn how to use it in order not to cut your fingers. Once you learn, it’s so useful and efficient that you’ll never want to go back to using a hand saw. And the same goes for machine learning. We want to help you understand and use machine learning correctly, fairly and safely.
Since machine learning is used in our everyday lives, it’s also important for everyone to understand how it impacts us. No matter whether you’re a coffee shop owner using machine learning to optimize the purchase of your beans based on seasonal trends, or your doctor diagnoses you with a disease with the help of this technology, it’s often crucial to understand why a machine learning model has produced the outcome it has. It’s also important for developers and decision-makers to be able to explain or present a machine learning model to people in order to do so. This is what we call “interpretability.”
How do you make machine learning models easier to understand and interpret?
There are many different ways to make an ML model easier to understand. One way is to make the model reflect how humans think from the start, and have the model "trained" to provide explanations along with predictions, meaning when it gives you an outcome, it also has to explain how it got there.
Another way is to try and explain a model after the training on data is done. This is something you can do when the model has been built to use input to provide an output from its own perspective, optimizing for prediction, without a clear “how” included. This means you're able to plug things into it and see what comes out, and that can give you some insight into how the model generally makes decisions, but you don't necessarily know exactly how specific inputs are interpreted by the model in specific cases.
One way to try and explain models after they’ve been trained is using low level features or high level concepts. Let me give you an example of what this means. Imagine a system that classifies pictures: you give it a picture and it says, “This is a cat.” A low level feature is when I then ask the machine which pixels mattered for that prediction, it can tell us if it was one pixel or the other, and we might be able to see that the pixels in question show the cat’s whiskers. But we might also see that it is a scattering of pixels that don’t appear meaningful to the human eye, or that it’s made the wrong interpretation. High level concepts are more similar to the way humans communicate with one another. Instead of asking about pixels, I’d ask, “Did the whiskers matter for the prediction? or the paws?” and again, the machine can show me what imagery led it to reach this conclusion. Based on the outcome, I can understand the model better. (Together with researchers from Stanford, we’ve published papers that go into further detail on this for those who are interested.)
Can machines understand some things that we humans can’t?
Yes! This is an area that I am very interested in myself. I am currently working on a way to showcase how technology can help humans learn new things. Machine learning technology is better at some things than we are; for example it can analyze and interpret data at a much larger scale than humans can. Leveraging this technology, I believe we can enlighten human scientists with knowledge they haven't previously been aware of.
What do you need to be careful of when you’re making conclusions based on machine learning models?
First of all, we have to be careful that human bias doesn't come into play. Humans carry biases that we simply cannot help and are often unaware of, so if an explanation is up to a human’s interpretation, and often it is, then we have a problem. Humans read what they want to read. Now, this doesn’t mean that you should remove humans from the loop. Humans communicate with machines, and vice versa. Machines need to communicate their outcomes in the form of a clear statement using quantitative data, not one that is vague and completely open for interpretation. If the latter happens, then the machine hasn’t done a very good job and the human isn’t able to provide good feedback to the machine. It could also be that the outcome simply lacks additional context only the human can provide, or that it could benefit from having caveats, in order for them to make an informed judgement about the results of the model.
What are some of the main challenges of this work?
Well, one of the challenges for computer scientists in this field is dealing with non mathematical objectives, which are things you might want to optimize for, but don’t have an equation for. You can’t always define what is good for humans using math. That requires us to test and evaluate methods with rigor, and have a table full of different people to discuss the outcome. Another thing has to do with complexity. Humans are so complex that we have a whole field of work - psychology - to study this. So in my work, we don't just have computational challenges, but also complex humans that we have to consider. Value-based questions such as “what defines fairness?” are even harder. They require interdisciplinary collaboration, and a diverse group of people in the room to discuss each individual matter.
What's the most exciting part?
I think interpretability research and methods are making a huge impact. Machine learning technology is a powerful tool that will transform society as we know it, and helping others to use it safely is very rewarding.
On a more personal note, I come from South Korea and grew up in circumstances where I feel I didn’t have too many opportunities. I was incredibly lucky to get a scholarship to MIT and come to the U.S. When I think about the people who haven't had these opportunities to be educated in science or machine learning, and knowing that this machine learning technology can really help and be useful to them in their everyday lives if they use it safely, I feel really motivated to be working on democratizing this technology. There's many ways to do it, and interpretability is one of the things that I can contribute with.