Posted by Sami Tolvanen, Staff Software Engineer, Android Security
Android's security model is enforced by the Linux kernel, which makes it a tempting target for attackers. We have put a lot of effort into hardening the kernel in previous Android releases and in Android 9, we continued this work by focusing on compiler-based security mitigations against code reuse attacks.
Google's Pixel 3 will be the first Android device to ship with LLVM's forward-edge Control Flow Integrity (CFI) enforcement in the kernel, and we have made CFI support available in Android kernel versions 4.9 and 4.14. This post describes how kernel CFI works and provides solutions to the most common issues developers might run into when enabling the feature.
Protecting against code reuse attacks
A common method of exploiting the kernel is using a bug to overwrite a function pointer stored in memory, such as a stored callback pointer or a return address that had been pushed to the stack. This allows an attacker to execute arbitrary parts of the kernel code to complete their exploit, even if they cannot inject executable code of their own. This method of gaining code execution is particularly popular with the kernel because of the huge number of function pointers it uses, and the existing memory protections that make code injection more challenging.
CFI attempts to mitigate these attacks by adding additional checks to confirm that the kernel's control flow stays within a precomputed graph. This doesn't prevent an attacker from changing a function pointer if a bug provides write access to one, but it significantly restricts the valid call targets, which makes exploiting such a bug more difficult in practice.
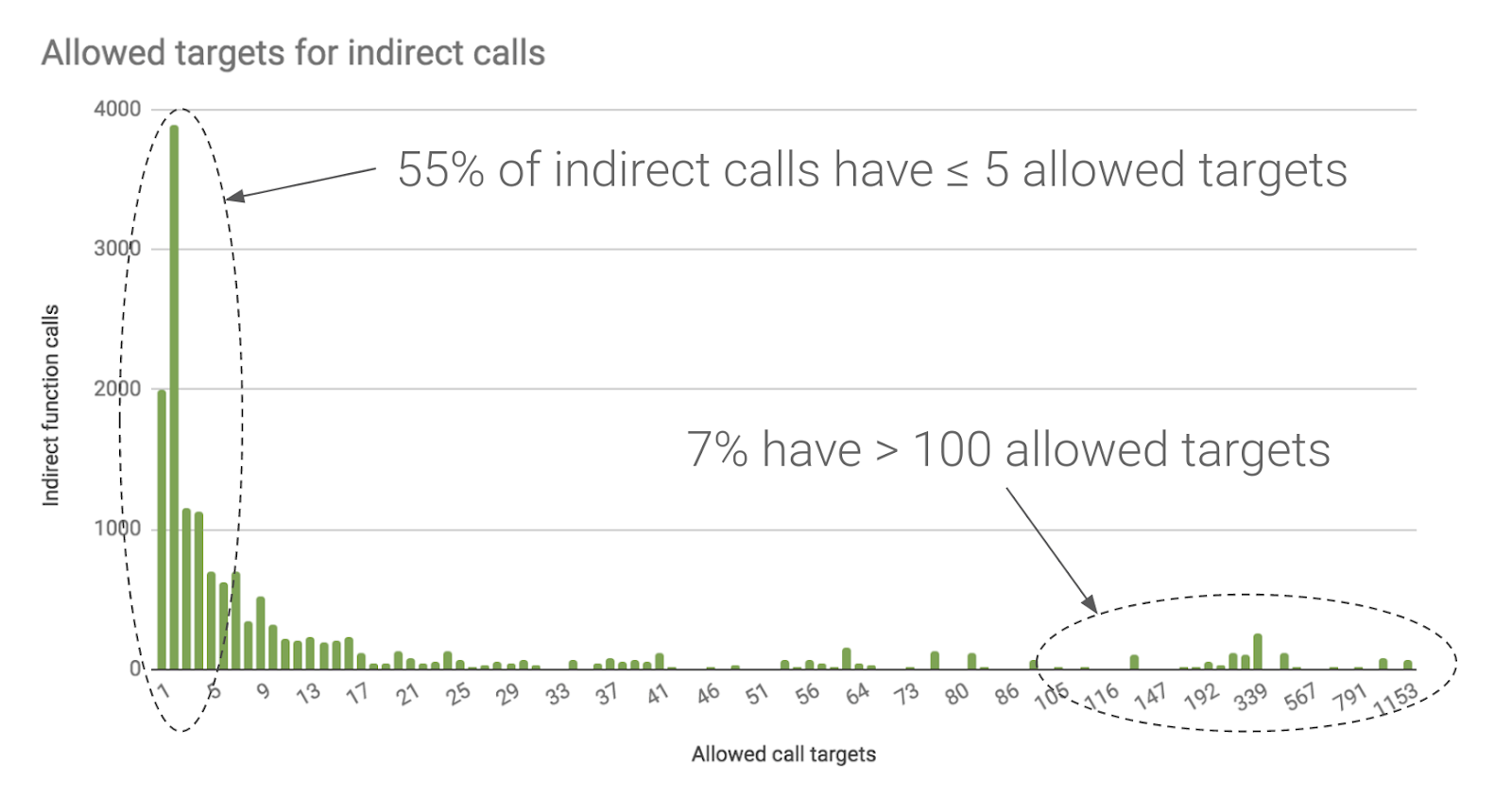
Figure 1. In an Android device kernel, LLVM's CFI limits 55% of indirect calls to at most 5 possible targets and 80% to at most 20 targets.
Gaining full program visibility with Link Time Optimization (LTO)
In order to determine all valid call targets for each indirect branch, the compiler needs to see all of the kernel code at once. Traditionally, compilers work on a single compilation unit (source file) at a time and leave merging the object files to the linker. LLVM's solution to CFI is to require the use of LTO, where the compiler produces LLVM-specific bitcode for all C compilation units, and an LTO-aware linker uses the LLVM back-end to combine the bitcode and compile it into native code.
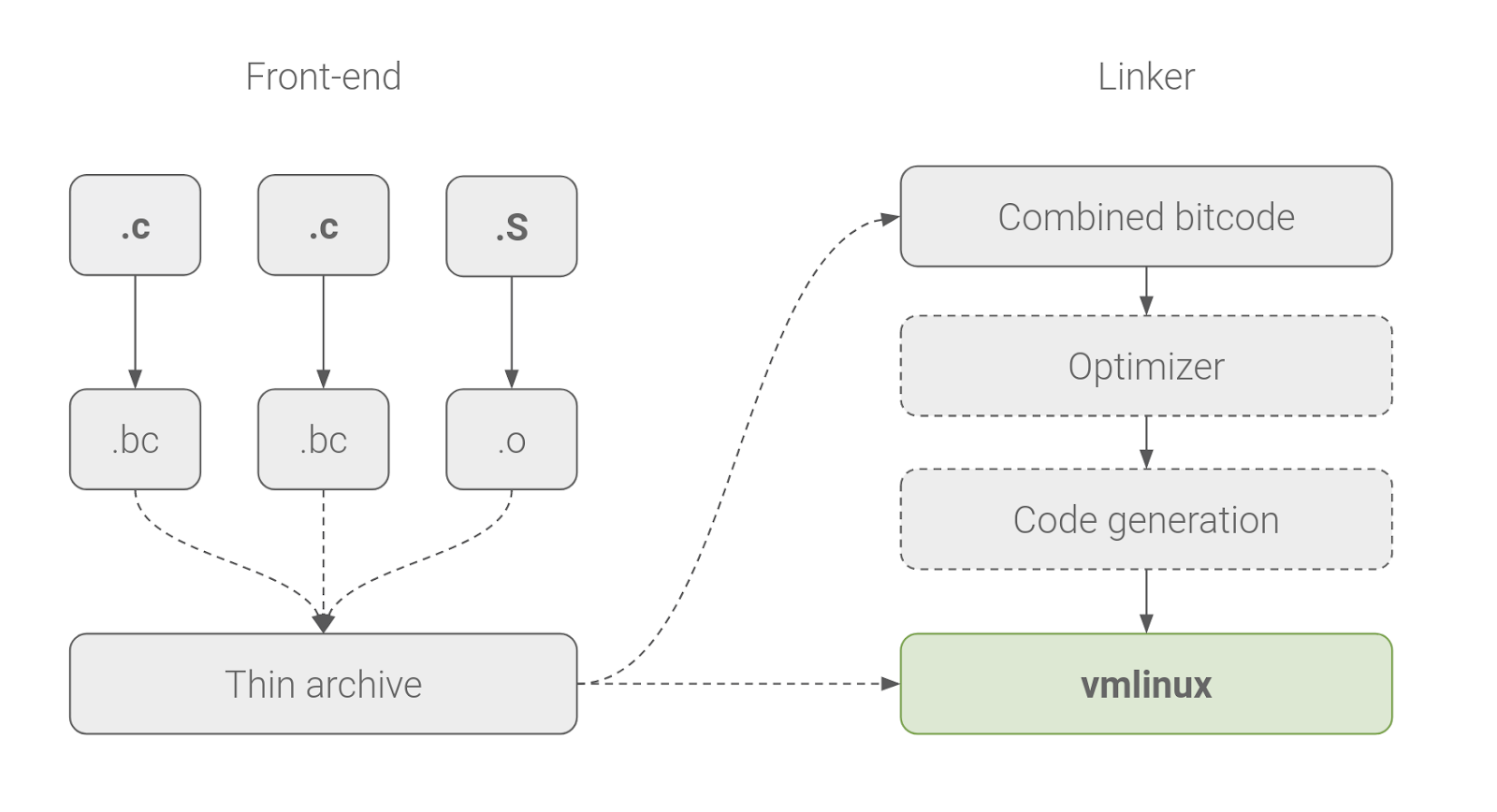
Figure 2. A simplified overview of how LTO works in the kernel. All LLVM bitcode is combined, optimized, and generated into native code at link time.
Linux has used the GNU toolchain for assembling, compiling, and linking the kernel for decades. While we continue to use the GNU assembler for stand-alone assembly code, LTO requires us to switch to LLVM's integrated assembler for inline assembly, and either GNU gold or LLVM's own lld as the linker. Switching to a relatively untested toolchain on a huge software project will lead to compatibility issues, which we have addressed in our arm64 LTO patch sets for kernel versions 4.9 and 4.14.
In addition to making CFI possible, LTO also produces faster code due to global optimizations. However, additional optimizations often result in a larger binary size, which may be undesirable on devices with very limited resources. Disabling LTO-specific optimizations, such as global inlining and loop unrolling, can reduce binary size by sacrificing some of the performance gains. When using GNU gold, the aforementioned optimizations can be disabled with the following additions to LDFLAGS:
LDFLAGS += -plugin-opt=-inline-threshold=0 \
-plugin-opt=-unroll-threshold=0
Note that flags to disable individual optimizations are not part of the stable LLVM interface and may change in future compiler versions.
Implementing CFI in the Linux kernel
LLVM's CFI implementation adds a check before each indirect branch to confirm that the target address points to a valid function with a correct signature. This prevents an indirect branch from jumping to an arbitrary code location and even limits the functions that can be called. As C compilers do not enforce similar restrictions on indirect branches, there were several CFI violations due to function type declaration mismatches even in the core kernel that we have addressed in our CFI patch sets for kernels 4.9 and 4.14.
Kernel modules add another complication to CFI, as they are loaded at runtime and can be compiled independently from the rest of the kernel. In order to support loadable modules, we have implemented LLVM's cross-DSO CFI support in the kernel, including a CFI shadow that speeds up cross-module look-ups. When compiled with cross-DSO support, each kernel module contains information about valid local branch targets, and the kernel looks up information from the correct module based on the target address and the modules' memory layout.
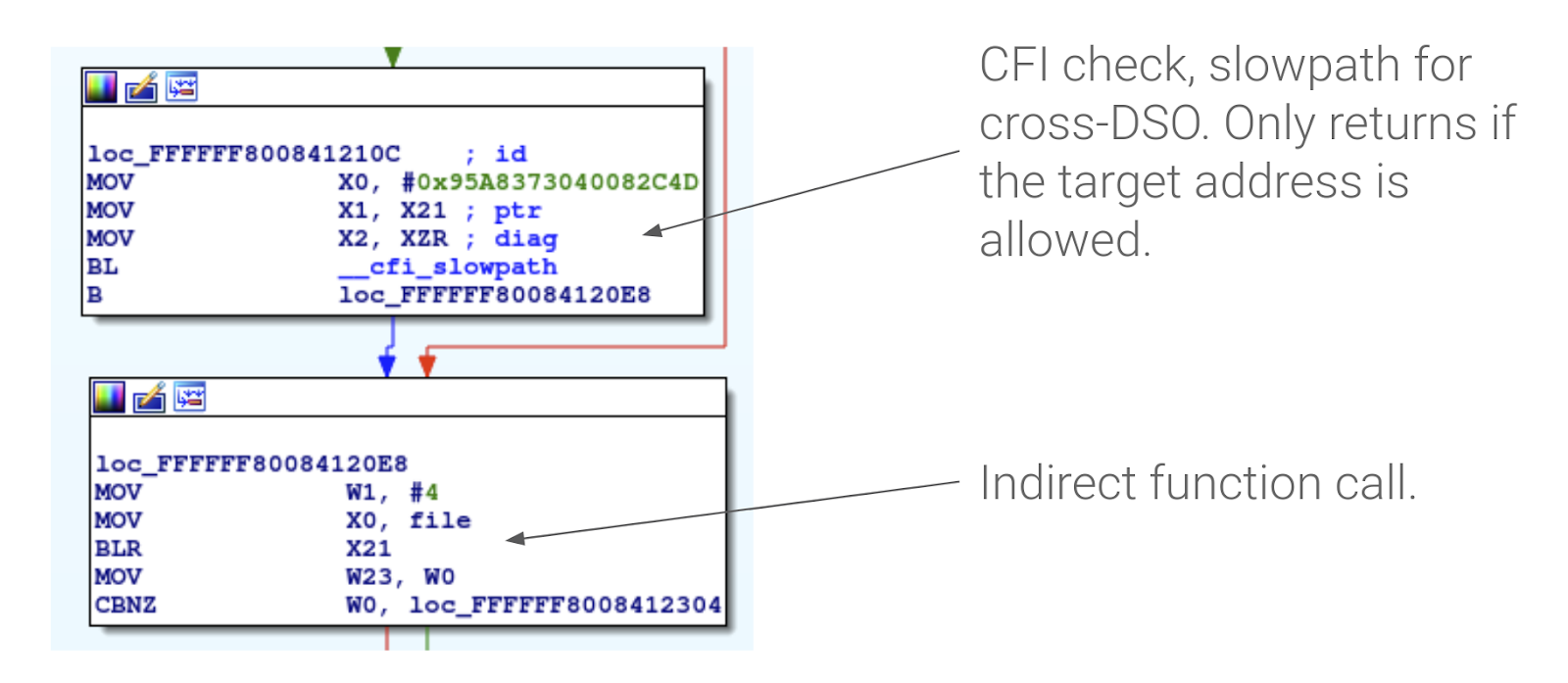
Figure 3. An example of a cross-DSO CFI check injected into an arm64 kernel. Type information is passed in X0 and the target address to validate in X1.
CFI checks naturally add some overhead to indirect branches, but due to more aggressive optimizations, our tests show that the impact is minimal, and overall system performance even improved 1-2% in many cases.
Enabling kernel CFI for an Android device
CFI for arm64 requires clang version >= 5.0 and binutils >= 2.27. The kernel build system also assumes that the LLVMgold.so plug-in is available in LD_LIBRARY_PATH. Pre-built toolchain binaries for clang and binutils are available in AOSP, but upstream binaries can also be used.
The following kernel configuration options are needed to enable kernel CFI:
CONFIG_LTO_CLANG=y
CONFIG_CFI_CLANG=y
Using CONFIG_CFI_PERMISSIVE=y may also prove helpful when debugging a CFI violation or during device bring-up. This option turns a violation into a warning instead of a kernel panic.
As mentioned in the previous section, the most common issue we ran into when enabling CFI on Pixel 3 were benign violations caused by function pointer type mismatches. When the kernel runs into such a violation, it prints out a runtime warning that contains the call stack at the time of the failure, and the call target that failed the CFI check. Changing the code to use a correct function pointer type fixes the issue. While we have fixed all known indirect branch type mismatches in the Android kernel, similar problems may be still found in device specific drivers, for example.
CFI failure (target: [<fffffff3e83d4d80>] my_target_function+0x0/0xd80):
------------[ cut here ]------------
kernel BUG at kernel/cfi.c:32!
Internal error: Oops - BUG: 0 [#1] PREEMPT SMP
…
Call trace:
…
[<ffffff8752d00084>] handle_cfi_failure+0x20/0x28
[<ffffff8752d00268>] my_buggy_function+0x0/0x10
…
Figure 4. An example of a kernel panic caused by a CFI failure.
Another potential pitfall are address space conflicts, but this should be less common in driver code. LLVM's CFI checks only understand kernel virtual addresses and any code that runs at another exception level or makes an indirect call to a physical address will result in a CFI violation. These types of failures can be addressed by disabling CFI for a single function using the __nocfi attribute, or even disabling CFI for entire code files using the $(DISABLE_CFI) compiler flag in the Makefile.
static int __nocfi address_space_conflict()
{
void (*fn)(void);
…
/* branching to a physical address trips CFI w/o __nocfi */
fn = (void *)__pa_symbol(function_name);
cpu_install_idmap();
fn();
cpu_uninstall_idmap();
…
}
Figure 5. An example of fixing a CFI failure caused by an address space conflict.
Finally, like many hardening features, CFI can also be tripped by memory corruption errors that might otherwise result in random kernel crashes at a later time. These may be more difficult to debug, but memory debugging tools such as KASAN can help here.
Conclusion
We have implemented support for LLVM's CFI in Android kernels 4.9 and 4.14. Google's Pixel 3 will be the first Android device to ship with these protections, and we have made the feature available to all device vendors through the Android common kernel. If you are shipping a new arm64 device running Android 9, we strongly recommend enabling kernel CFI to help protect against kernel vulnerabilities.
LLVM's CFI protects indirect branches against attackers who manage to gain access to a function pointer stored in kernel memory. This makes a common method of exploiting the kernel more difficult. Our future work involves also protecting function return addresses from similar attacks using LLVM's Shadow Call Stack, which will be available in an upcoming compiler release.