Posted by Aleem Mawani, Co-Founder, Streak.com
Editor's note: Today, we hear from Aleem Mawani, co-founder of Streak.com, a Google Cloud Platform customer whose customer relationship management (CRM) for Google Apps is built entirely on top of Google products: Gmail, Google App Engine and Google Cloud Datastore. Read on to learn how Streak added advanced functionality to the Cloud Datastore object storage system
Streak is a full blown CRM built directly into Gmail. We’re built on
Google Cloud Platform (most heavily on
Google App Engine) and we store terabytes of user data in
Google Cloud Datastore. It’s our primary database, and we’ve been happy with its scalability, consistent performance and zero-ops management. However, we did want more functionality in a few areas. Instead of overwriting database entities with their new content whenever a user updated their data, we wanted to store every version of those entities and make them easy to access. Specifically, we wanted a way to make all of our data immutable.
In this post, I’ll go over why you might want to use immutable entities, and our approach for implementing them on top of Cloud Datastore.
There are a few reasons why we thought immutable entities were important.
- We wanted an easy way to implement a newsfeed-style UI. Typical newsfeeds show how an entity has changed over time in a graphical format to users. Traditionally we stored separate side entities to record the deltas between different versions of a single entity. Then we’d query for those side entities to render a newsfeed. Designing these side entities was error prone and not easily maintainable. For example, if you added a new property to your entity, you would need to remember to also add that to the side entities. And if you forgot to add certain data to the side entities, there was no way to reconstruct that later down the line when you did need it — the data was gone forever.
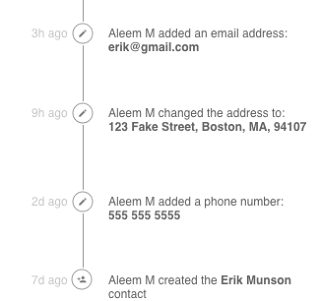
The "Contact" entity stores data about users’ contacts. Because it’s implemented as an immutable entity, it's easy to generate a historical record of how that contact has changed over time. |
- Having immutable entities allows us to recover from user errors very easily. Users can rollback their data to earlier versions or even recover data they may have accidentally deleted (see how we implemented deletion below)1.
- Potentially easier debugging. It’s often useful to see how an entity changed over time and got into its current state. We can also run historical queries on the number of changes to an entity - useful for user behaviour analysis or performance optimization.
Some context
Before we go into our implementation of immutable entities on the Cloud Datastore, we need to understand some of the basics of how the datastore operates. If you’re already familiar with the Cloud Datastore, feel free to skip this section.
You can think of the Cloud Datastore as a key-value store. A value, called an entity in the datastore, is identified by its key, and the entity itself is just a bag of properties. There's no enforcement of a schema on all entities in a table so the properties of two entities need not be the same.
The database also supports basic queries on a single table
— there are no joins or aggregation, just simple table scans for which an index can be built. While this may seem limiting, it enables fast and consistent query performance because you will typically
denormalize your data.
The most important property of Cloud Datastore for our implementation of immutable entities is “entity groups.” Entity groups are groups of entities for which you get two guarantees:
- Queries that are restricted to a single entity group get consistent results. This means that a write immediately followed by a query will have results that are guaranteed to reflect the changes made by the write. Conversely, if your query is not limited to a single entity group you may not get consistent results (stale data).
- Multi-entity transactions can only be applied within a single entity group (this was recently improved — Cloud Datastore now supports cross entity group transactions but limits the number of entity groups involved to 25).
Both of these facts will be important in our implementation. For more details on how the Cloud Datastore itself works, see the
documentation.
How we implemented immutable entities
We needed a way to store every change we made to a single entity while supporting common operations for entities: get, delete, update, create and query. The overall strategy we took was to utilize two levels of abstraction
— a "datastore entity" and a "logical entity." We used individual "datastore entities" to represent individual versions of a "logical entity." Users of our API would only interact with logical entities and each logical entity would have a key to identify it and support the common get, create, update, delete and query operations. These logical entities would be backed by actual datastore entities comprising the different versions of that logical entity. The most recent, or tip, version of the datastore entities represented the current value of the logical entity. First let’s start with what the data model looks like. Here’s how we designed our entity:
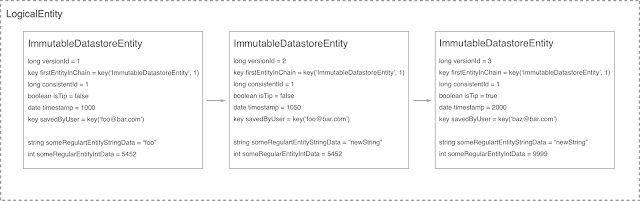 |
(click to enlarge) |
The way this works is that we always store a new datastore entity every time the user would like to make a change to the entity. The most recent datastore entity has the
isTip
value set to true and the rest don’t. We'll use this field later to query for a particular logical entity by getting the tip data store entity. This query is fast in the data store because all queries are required to have indexes. We also store the timestamp for when each datastore entity was created.
The
versionId
field is a globally unique identifier for each datastore entity. These IDs are automatically assigned by Cloud Datastore when we store the entity.
The
consistentId
identifies a logical entity
— it's the ID we can give to users of this API. All of the datastore entities in a logical entity have the same consistent ID. We picked the consistent ID of the logical entity to be equal to the ID of the first datastore entity in the chain. This is somewhat arbitrary, and we could have picked any unique identifier, but since the low level Cloud Datastore API gives us a unique ID for every datastore entity, we decided to use the first one as our consistent ID.
The other interesting part of this data model is the
firstEntityInChain
field. What's not shown in the diagram is that every datastore entity has its parent (the parent determines the entity group) set to the first datastore entity in the chain. It's important that all the datastore entities in the chain (including the first one) have the same parent and are thus in the same entity group so that we can perform consistent queries. You’ll see why these are needed below.
Here’s the same immutable entity defined in code. We use the awesome
Objectify library with the Cloud Datastore and these snippets do make use of it.
public class ImmutableDatastoreEntity {
@Id
Long versionId;
@Parent
Key<T> firstEntityInChain;
protected Long consistentId;
protected boolean isTip;
Key<User> savedByUser;
}
So how do we perform common operations on logical entities given that they are backed by datastore entities?
Performing creates
When creating a logical entity, we just need to create a single new datastore entity and use the Cloud Datastore’s ID allocation to set the
versionId
field and the
consistentId
field to the same value. We also set the parent key (
firstEntityInChain
) to point to itself. We also have to set
isTip
to true so we can query for this entity later. Finally we set the timestamp and the creator of the datastore entity and persist the entity to Cloud Datastore.
ImmutableDatastoreEntity entity = new ImmutableDatastoreEntity();
entity.setVersionId(DAO.allocateId(this.getClass()));
entity.setConsistentId(entity.getVersionId());
entity.setFirstEntityInChain((Key<T>) Key.create(entity.getClass(), entity.versionId));
entity.setTip(true);
Performing updates
To update a logical entity with new data, we first need to fetch the most recent datastore entity in the chain (we describe how in the "get" section below). We then create a new datastore entity and set the consistentId
and firstEntityInChain
to that of the previous datastore entity in the chain. We set isTip
to true on the new datastore entity and set it to false on the old datastore entity (note this is the only instance in which we modify an existing entity so we aren’t 100% immutable).
We finally fill in the timestamp and user keys fields, and we’re ready to store the new datastore entity. Two important points on this: for the new datastore entity, we can let the datastore automatically allocate the ID when storing the entity (because we don’t need to use it anywhere else). Second, it's incredibly important that we fetch the existing datastore entity and store both the new and old datastore entity in the same transaction. Without this, our data could become internally inconsistent.
// start transaction
ImmutableDatastoreEntity oldVersion = getImmutableEntity(immutableId)
oldVersion.setTip(false);
ImmutableDatastoreEntity newVersion = oldVersion.clone();
// make the user edits needed
newVersion.setVersionId(null);
newVersion.setConsistentId(this.getConsistentId());
newVersion.setFirstEntityInChain(oldVersion.getFirstEntityInChain());
// .clone also performs the last two lines but just to be explicit this, just fyi
newVersion.setTip(true);
ofy().save(oldVersion, newVersion).now();
// end transaction
Performing gets
Performing a get actually requires us to do a query operation to the datastore because we need to find the datastore entity that has a certain consistentId
AND has isTip
set to true. This entity will represent the logical entity. Because we want the query to be consistent, we must perform an ancestor query (i.e., tell Cloud Datastore to limit the query to a certain entity group). This only works because we ensured that all datastore entities for a particular logical entity are part of the same entity group.
This query should only ever return one result — the datastore entity that represents the logical entity.
Key ancestorKey = KeyFactory.createKey(ImmutableDatastoreEntity.class, consistentId);
ImmutableDatastoreEntity e = ofy().load()
.kind(ImmutableDatastoreEntity.class)
.filter("consistentId", consistentId)
.filter("isTip", true)
.ancestor(ancestorKey) // this limits our query to just the 1 entity group
.list()
.first();
Performing deletes
In order to delete logical entities, all we need to do is set the isTip
of the most recent datastore entity to false. By doing this we ensure that the "get" operation described above no longer returns a result, and similarly, queries such as those described below continue to operate.
// wrap block in transaction
ImmutableDatastoreEntity oldVersion = getImmutableEntity(immutableId);
oldVersion.setTip(false);
ofy().save(oldVersion, newVersion).now();
Performing queries
We need to be able to perform queries across all logical entities. However, when querying every datastore entity, we need to modify our queries so that they only consider the tip datastore entity of each logical entity (unless you explicitly want to find old versions of the data). To do this, we need to add an extra filter to our queries to just consider tip entities. One important thing to note is that we cannot do consistent queries in this case because we cannot guarantee that all the results will be in the same entity group (in fact we know for certain they are not if there are multiple results)
List<ImmutableDatastoreEntity> results = ofy().load()
.kind(ImmutableDatastoreEntity.class)
.filter("isTip", true)
.filter(/** apply other filters here */)
.list();
Performing newsfeed queries
One of our goals was to be able to show how a logical entity has changed over time, so we must be able to query for all datastore entities in a chain. Again, this is a fairly straightforward query
— we can just query by the
consistentId
and order by the timestamp. This will give us all versions of the logical entity. We can diff each datastore entity against the previous datastore entity to generate the data needed for a newsfeed.
Key ancestorKey = KeyFactory.createKey(ImmutableDatastoreEntity.class, consistentId);
List<ImmutableDatastoreEntity> versions = ofy().load()
.kind(ImmutableDatastoreEntity.class)
.filter("consistentId", consistentId)
.ancestor(ancestorKey)
.list();
Downsides
Using the design described above, we were able to achieve our goal of having roughly immutable entities that are easy to debug and make it easy to build newsfeed-like features. However, there are some drawbacks to this method:
- We need to do a query any time we need to get an entity. In order to get a specific logical entity, we actually need to perform a query as described above. On Cloud Datastore, this is a slower operation than a traditional "get" by key. Additionally, Objectify offers built-in caching, which also can’t be used when trying to get one of our immutable entities (because Objectify can’t cache queries). To address this, we’ll need to implement our own caching in memcache if performance becomes an issue.
- There's no method to do a batch get of entities. Because each query must be restricted to a single entity group for consistency, we can’t fetch the tip datastore entity for multiple logical entities with just one datastore operation. To address this, we perform multiple asynchronous queries and wait for all to finish. This isn’t ideal or clean, but it works fairly well in practice. Remember that on App Engine there's a limit of 30 outstanding RPCs when making concurrent RPC calls, so this only takes you so far.
- High implementation cost for the first entity. We abstracted most of the design described above so that future immutable entities would be cheap for us to implement, however, the first entity wasn’t trivial to implement. It took us some time to iron out all the kinks, so it’s definitely only worth doing this if you very much need immutability or if you’ll be spreading the implementation cost across many use cases.
- Entities are never actually deleted. By design, we don’t delete immutable entities. However, from a user perspective, they may have the expectation that once they delete something in our app, we actually delete the data. This also might be the expectation in some regulated industries (i.e., healthcare). For our use case, it wasn't necessary, but you may want to develop a system that maps over your dataset and finds fully deleted logical entities and deletes all of the datastore entities representing them in some batch task periodically.
Next steps
We’ve only been running with immutable entities in production for a little while, and it remains to be seen what problems we’ll face. And as we implement a few more of our datasets as immutable entities, it will become clear whether the implementation costs were worth the effort. Subscribe to
our blog to get updates.
If this sort of data infrastructure floats your boat, definitely reach out to us as we have several openings on our backend team. Check out our
job postings for more info.
Discuss on
Hacker News
1This is very similar to the idea of MVCC (https://en.wikipedia.org/wiki/Multiversion_concurrency_control) which is how many modern databases implement transactions and rollback.